我們這一年來做的一些工作(總結見文章最下方)現在差不多形成了一個較完善的計算機視覺工具鏈——CVChain。我們這一年來做的一些工作(總結見文章最下方)現在差不多形成了一個較完善的計算機視覺工具鏈——CVChain。
縱向上它涵蓋了一個計算機視覺任務的生命周期:數據分析與模型選型、模型訓練、發現模型存在的問題并優化、模型加速、模型SDK編寫;橫向上它包含了計算機視覺中三個基本任務:分類、語義分割、目標檢測;與此同時它還總結了計算機視覺入門到進階的學習框架。一言以蔽之:有了CVChain,媽媽再也不用擔心我搞不定計算機視覺!
CVChain是我們平常做項目或者打比賽過程中打磨出來的,它們可以滿足計算機視覺算法工程師日常大部分需求,比如:
1.剛踏入計算機視覺領域,不知道從何學起,需要一張學習的地圖:
https://github.com/mileistone/study_resources/blob/master/modeling/learning_framework/learning_framework_general.md
部分示例
帶著自己一步一步領略計算機視覺的風采;
2.已經成為一名合格的計算機視覺算法工程師,開始接任務。當任務來了,需要分析數據分析數據以進行模型選型、模型超參的初步設定;
https://github.com/Media-Smart/volkscv/tree/master/volkscv/analyzer/statistics
3.模型確定后,得訓練模型(可能涉及到分類、語義分割、文字識別、目標檢測等等),這個時候需要一個趁手的訓練工具;
分類:
https://github.com/Media-Smart/vedacls
語義分割
https://github.com/Media-Smart/vedaseg
示例
文字識別
https://github.com/Media-Smart/vedastr
示例
目標檢測
https://github.com/Media-Smart/vedadet
4.模型訓練完之后,效果不夠好,我們需要把FP、FN打印出來,分析模型存在的問題;
https://github.com/Media-Smart/volkscv/tree/master/volkscv/analyzer/visualization
5.模型訓練好之后,需要將模型轉換為應用并進行部署,這里需要用TensorRT對模型進行加速,然后根據業務需求編寫Python前端或者C++前端的SDK;
加速
https://github.com/Media-Smart/volksdep
Python前端
https://github.com/Media-Smart/flexinfer
示例
C++前端
https://github.com/Media-Smart/cheetahinfer
6.計算機行業競爭激烈,平常得抽空加強學習,無論是工程、模型還是算法方面,都需要持續不斷學習,把自己訓練為一名六邊形戰士。
工程
https://github.com/mileistone/study_resources/tree/master/engineering
模型
https://github.com/mileistone/study_resources/tree/master/modeling
算法
https://github.com/mileistone/study_resources/tree/master/modeling/optimization_and_generalization
上述的“2、數據分析”提供以下功能。
1、瀏覽圖片和標注
比如分類、目標檢測、語義分割等等,這可以幫助我們對數據有一個感性的認識,可以定性出來這個任務有哪些挑戰。
2、圖片和標注分析
比如圖片大小分布,圖片長寬比分布,圖片中GT框數量分布,GT框長寬分布等等,這可以讓我們對數據有一些理性的認識,讓我們可以定量這個任務存在的挑戰。
3、打印模型預測結果中的FP、FN
比如分類。
比如目標檢測。
比如語義分割。
打印FP、FN可以讓我們發現模型存在的問題,進而有助于我們分析問題、定位問題直至解決問題。
4、anchor分析
比如GT匹配上的anchor數量分布,GT與匹配上anchor的IoU分布等等。這有助于我們設計出更好的anchor策略,比如anchor應該放在哪幾層,每一層anchor數量應該設置多少,對應的大小和長寬比是多少,以及label assignment該怎么做等等。
匯總
https://github.com/Media-Smart/vedaseg
https://github.com/Media-Smart/vedastr
https://github.com/Media-Smart/vedacls
https://github.com/Media-Smart/vedadet
Media-Smart/volksdep,https://github.com/Media-Smart/volksdep
Media-Smart/flexinfer,https://github.com/Media-Smart/flexinfer
https://github.com/Media-Smart/cheetahinfer
https://github.com/Media-Smart/volkscv
https://github.com/mileistone/study_resources
- 數據分析 - [volkscv](https://github.com/Media-Smart/volkscv/tree/master/volkscv/analyzer/) - 數據瀏覽 -> 獲取感性認識 - 圖片、標注 - 數據統計 -> 獲取理性認識 - 圖片統計 - 大小 - 長寬比 - 等等 - 標注統計 - 類別 - 各個類別有多少實例 - 等等 - GT框 - 大小 - 長寬比 - 等等 - anchor分析 - GT掛上anchor的數量分布 - GT與掛上anchor的IoU分布- 模型訓練 - [vedaseg](https://github.com/Media-Smart/vedaseg) - semantic segmentation - [vedastr](https://github.com/Media-Smart/vedastr) - scene text recognition - [vedacls](https://github.com/Media-Smart/vedacls) - classification - [vedadet](https://github.com/Media-Smart/vedadet) - object detection - 應用部署 - [volksdep](https://github.com/Media-Smart/volksdep) - increase efficiency and decrease latency - convert PyTorch,ONNX model to TensorRT engine - [flexinfer](https://github.com/Media-Smart/flexinfer) -> Python front end SDK based on TensorRT engine - classification - semantic segmentation - scene text recognition - object detection - [cheetahinfer](https://github.com/Media-Smart/cheetahinfer) -> C++ front end SDK based on TensorRT engine - classification - semantic segmentation - object detection- 學習資源 - [學習框架]
(https://github.com/mileistone/study_resources/tree/master/modeling/learning_framework) - 知識點 - 相關課程與書籍 - 基礎 - [工程](https://github.com/mileistone/study_resources/tree/master/engineering) - 編程語言 - Python - C++ - 軟件工程 - 設計模式 - 操作系統 - Linux - Bash - Vim - 編譯工具鏈 - [模型](https://github.com/mileistone/study_resources/tree/master/modeling) - 內容 - 機器學習 - 深度學習 - 計算機視覺 - 形式 - 課程 - 書籍 - 論文 - [算法](https://github.com/mileistone/study_resources/tree/master/modeling/optimization_and_generalization) - 凸優化 - 數值優化
責任編輯:xj
原文標題:CVChain:一條用視覺競賽和項目經驗打磨出的計算機視覺完整工具鏈
文章出處:【微信公眾號:新機器視覺】歡迎添加關注!文章轉載請注明出處。
-
CV
+關注
關注
0文章
53瀏覽量
16861 -
計算機視覺
+關注
關注
8文章
1698瀏覽量
45994
原文標題:CVChain:一條用視覺競賽和項目經驗打磨出的計算機視覺完整工具鏈
文章出處:【微信號:vision263com,微信公眾號:新機器視覺】歡迎添加關注!文章轉載請注明出處。
發布評論請先 登錄
相關推薦
計算機視覺的五大技術
計算機視覺的工作原理和應用
機器視覺與計算機視覺的區別
本源超導量子計算機自主制造鏈11類產品系列之九: 中國自主量子計算編程生態工具鏈
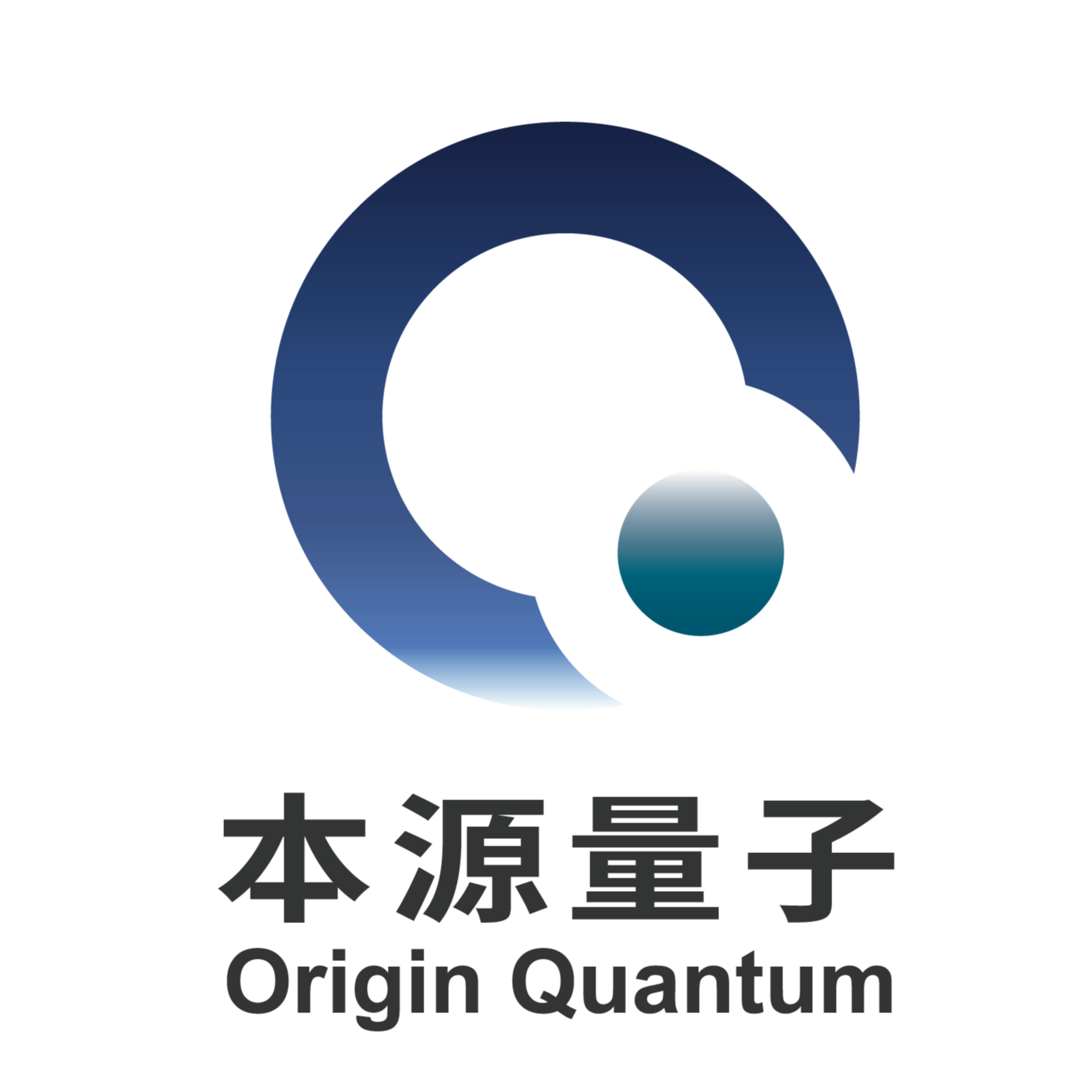
評論