在材料科學領域,制備具有特定光學性能納米材料的方法極為重要。近年來,機器學習方法已用于解決材料科學中的眾多方面,例如藥物發現、醫學成像、材料合成、功能分子生成和材料降解等等。然而,目前機器學習的算法大多是基于計算模擬的數據或從文獻中收集的實驗數據而開發的,這導致輸出的目標材料難以在實驗上合成,限制了其實際應用。
來自新加坡國立大學化學與生物分子工程系Xiaonan Wang教授領銜的團隊,提出了一個兩步式理論框架,以實現機器學習驅動的高通量微流控平臺,并用于快速生產具有所需吸收光譜的銀納米顆粒。作者結合高斯過程的貝葉斯優化算法與深度神經網絡,并與高通量實驗合成一個循環,有效地優化了銀納米顆粒的合成。此外,此框架還訓練了一種可轉移算法,可以使用最終的深度神經網絡進行逆設計,以合成具有不同光學特性的納米粒子。作者所開發的方法適用于微流體高通量實驗回路中其他材料的合成,同時還適用于其他類型的高通量實驗平臺,具有重要的應用價值。
該文近期發表于npj Computational Materials7: 55(2021),英文標題與摘要如下,點擊左下角“閱讀原文”可以自由獲取論文PDF。
Two-step machine learning enables optimized nanoparticle synthesis
Flore Mekki-Berrada, Zekun Ren, Tan Huang, Wai Kuan Wong, Fang Zheng, Jiaxun Xie, Isaac Parker Siyu Tian, Senthilnath Jayavelu, Zackaria Mahfoud, Daniil Bash, Kedar Hippalgaonkar, Saif Khan, Tonio Buonassisi, Qianxiao Li & Xiaonan Wang
In materials science, the discovery of recipes that yield nanomaterials with defined optical properties is costly and time-consuming. In this study, we present a two-step framework for a machine learning-driven high-throughput microfluidic platform to rapidly produce silver nanoparticles with the desired absorbance spectrum. Combining a Gaussian process-based Bayesian optimization (BO) with a deep neural network (DNN), the algorithmic framework is able to converge towards the target spectrum after sampling 120 conditions. Once the dataset is large enough to train the DNN with sufficient accuracy in the region of the target spectrum, the DNN is used to predict the colour palette accessible with the reaction synthesis. While remaining interpretable by humans, the proposed framework efficiently optimizes the nanomaterial synthesis and can extract fundamental knowledge of the relationship between chemical composition and optical properties, such as the role of each reactant on the shape and amplitude of the absorbance spectrum.
文章出處:【微信公眾號:知社學術圈】
責任編輯:gt
-
神經網絡
+關注
關注
42文章
4777瀏覽量
100995 -
機器學習
+關注
關注
66文章
8434瀏覽量
132873
原文標題:npj: 納米材料的精確合成—機器學習的高通量微流控平臺
文章出處:【微信號:zhishexueshuquan,微信公眾號:知社學術圈】歡迎添加關注!文章轉載請注明出處。
發布評論請先 登錄
相關推薦
高通量生物分析技術之微流控芯片
S型微流控芯片的優勢
TensorFlow與PyTorch深度學習框架的比較與選擇
中國移動浙江公司攜手華為完成5G-A高通量UPF全球現網首呼
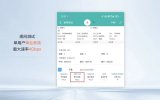
賦能產業互聯網,高通量計算讓世界更高效!
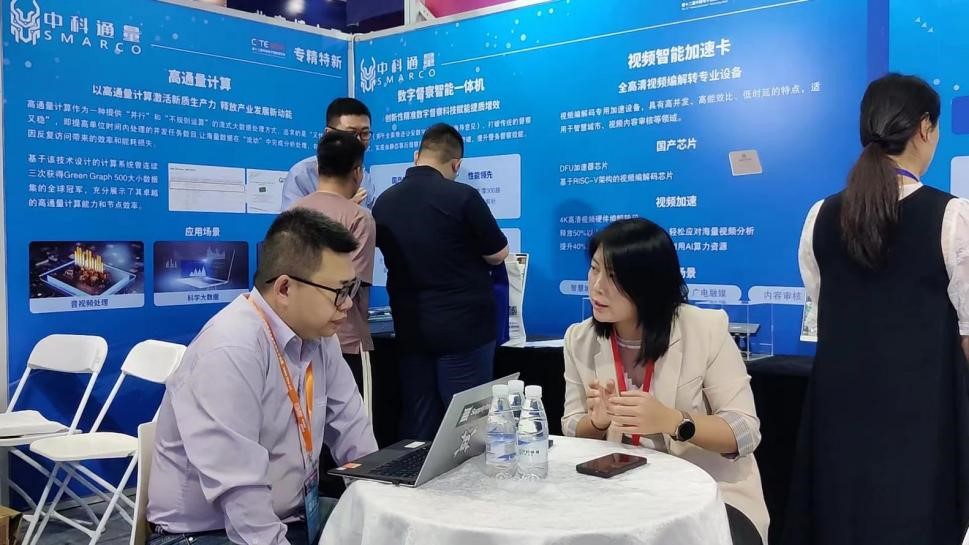
評論