機器學習中一個常見問題是判定與數(shù)據(jù)交互的最佳方式。
在本文中,我們將提供一種高效方法,用于完成數(shù)據(jù)的交互、組織以及最終變換(預處理)。隨后,我們將講解如何在訓練過程中正確地把數(shù)據(jù)輸入給模型。
PyTorch 框架將幫助我們實現(xiàn)此目標,我們還將從頭開始編寫幾個類。PyTorch 可提供更完整的原生類,但創(chuàng)建我們自己的類可幫助我們加速學習。
第 1 部分:原始數(shù)據(jù)和數(shù)據(jù)集
首先我們把尚未經(jīng)過組織的所有樣本稱為“原始數(shù)據(jù)”。
把“數(shù)據(jù)集”定義為現(xiàn)成可用的數(shù)據(jù),即含標簽以及基本函數(shù)接口(以便于使用原始數(shù)據(jù)信息)的原始數(shù)據(jù)。
此處我們使用一種簡單的原始數(shù)據(jù)形式:1 個包含圖像和標簽的文件夾。
但此方法可擴展至任意性質(zhì)的樣本(可以是圖片、錄音、視頻等)以及包含標簽的文件。
標簽文件中的每一行都用于描述 1 個樣本和相關(guān)標簽,格式如下:
file_sample_1 label1
file_sample_2 label2
file_sample_3 label3
(。..)
當能夠完成一些基本信息查詢(已有樣本數(shù)量、返回特定編號的樣本、預處理每個樣本等)時,說明我們已從原始數(shù)據(jù)集創(chuàng)建了 1 個數(shù)據(jù)集。
此方法基于面向?qū)ο?a target="_blank">編程以及創(chuàng)建用于數(shù)據(jù)處理的 “類”。
對于一組簡單的圖像和標簽而言,此方法可能看上去略顯殺雞用牛刀(實際上,此用例通常是通過創(chuàng)建分別用于訓練、驗證和測試的獨立文件夾來進行處理的)。但如果要選擇標準交互方法,則此方法將來可復用于多種不同用例,以節(jié)省時間。
在 Python 中處理數(shù)據(jù)
在 Python 中所有一切都是對象:整數(shù)、列表、字典都是如此。
構(gòu)建含標準屬性和方法的“數(shù)據(jù)集”對象的原因多種多樣。我認為,代碼的精致要求就足以合理化這一選擇,但我理解這是品味的問題。可移植性、速度和代碼模塊化可能是最重要的原因。
在許多示例以及編碼書籍中,我發(fā)現(xiàn)了面向?qū)ο蟮木幋a(尤以類為甚)的其它有趣的功能和優(yōu)勢,總結(jié)如下:
? 類可提供繼承
? 繼承可提供復用
? 繼承可提供數(shù)據(jù)類型擴展
? 繼承支持多態(tài)現(xiàn)象
? 繼承是面向?qū)ο蟮木幋a的特有功能
■輸入 [1]:
import torch
from torchvision import transforms
to_tensor = transforms.ToTensor()
from collections import namedtuple
import functools
import copy
import csv
from PIL import Image
from matplotlib import pyplot as plt
import numpy as np
import os
import datetime
import torch.optim as optim
在我們的示例中,所有原始樣本都存儲在文件夾中。此文件夾的地址在 raw_data_path 變量中聲明。
■輸入 [2]:
raw_data_path = ‘。/raw_data/data_images’
構(gòu)建模塊
數(shù)據(jù)集接口需要一些函數(shù)和類。數(shù)據(jù)集本身就是一個對象,因此我們將創(chuàng)建 MyDataset 類來包含所有重要函數(shù)和變量。
首先,我們需要讀取標簽文件,然后可對樣本在其原始格式(此處為 PIL 圖像)以及最終的張量格式應(yīng)用某些變換。
我們需要使用以下函數(shù)來讀取 1 次標簽文件,然后創(chuàng)建包含所有樣本名稱和標簽的元組。
內(nèi)存中緩存可提升性能,但如果標簽文件發(fā)生更改,請務(wù)必更新緩存內(nèi)容。
■ 輸入 [113]:
DataInfoTuple = namedtuple(‘Sample’,‘SampleName, SampleLabel’)
def myFunc(e):
return e.SampleLabel
# in memory caching decorator: ref https://dbader.org/blog/python-memoization
@functools.lru_cache(1)
def getSampleInfoList(raw_data_path):
sample_list = []
with open(str(raw_data_path) + ‘/labels.txt’, mode = ‘r’) as f:
reader = csv.reader(f, delimiter = ‘ ’)
for i, row in enumerate(reader):
imgname = row[0]
label = int(row[1])
sample_list.append(DataInfoTuple(imgname, label))
sample_list.sort(reverse=False, key=myFunc)
# print(“DataInfoTouple: samples list length = {}”.format(len(sample_list)))
return sample_list
如需直接變換 PIL 圖像,那么以下類很實用。
該類僅含 1 種方法:resize。resize 方法能夠改變 PIL 圖像的原始大小,并對其進行重新采樣。如需其它預處理(翻轉(zhuǎn)、剪切、旋轉(zhuǎn)等),需在此類種添加方法。
當 PIL 圖像完成預處理后,即可將其轉(zhuǎn)換為張量。此外還可對張量執(zhí)行進一步的處理步驟。
在以下示例種,可以看到這兩種變換:
■ 輸入 [4]:
class PilTransform():
“”“generic transformation of a pil image”“”
def resize(self, img, **kwargs):
img = img.resize(( kwargs.get(‘width’), kwargs.get(‘height’)), resample=Image.NEAREST)
return img
# creation of the object pil_transform, having all powers inherited by the class PilTransform
pil_transform = PilTransform()
以下是類 PilTransform 的實操示例:
■ 輸入 [5]:
path = raw_data_path + “/img_00000600.JPEG”
print(path)
im1 = Image.open(path, mode=‘r’)
plt.imshow(im1)
。/raw_data/data_images/img_00000600.JPEG
■ 輸出 [5]:
■ 輸入 [6]:
im2 = pil_transform.resize(im1, width=128, height=128)
# im2.show()
plt.imshow(im2)
■ 輸出 [6]:
最后,我們定義一個類,用于實現(xiàn)與原始數(shù)據(jù)的交互。
類 MyDataset 主要提供了 2 個方法:
__len__ 可提供原始樣本的數(shù)量。
__getitem__ 可使對象變?yōu)榭傻愋停磸埩扛袷椒祷卣埱蟮臉颖荆ㄒ淹瓿深A處理)。
__getitem__ 步驟:
1) 打開來自文件的樣本。
2) 按樣本的原始格式對其進行預處理。
3) 將樣本變換為張量。
4) 以張量格式對樣本進行預處理。
此處添加的預處理僅作為示例。
此類可對張量進行歸一化(求平均值和標準差),這有助于加速訓練過程。
請注意,PIL 圖像由范圍 0-255 內(nèi)的整數(shù)值組成,而張量則為范圍 0-1 內(nèi)的浮點數(shù)矩陣。
該類會返回包含兩個元素的列表:在位置 [0] 返回張量,在位置 [1] 返回包含 SampleName 和 SampleLabel 的命名元組。
■ 輸入 [109]:
class MyDataset():
“”“Interface class to raw data, providing the total number of samples in the dataset and a preprocessed item”“”
def __init__(self,
isValSet_bool = None,
raw_data_path = ‘。/’,
SampleInfoList = DataInfoTuple,norm = False,
resize = False,
newsize = (32, 32)
):
self.raw_data_path = raw_data_path
self.SampleInfoList = copy.copy(getSampleInfoList(self.raw_data_path))
self.isValSet_bool = isValSet_bool
self.norm = norm
self.resize = resize
self.newsize = newsize
def __str__(self):
return ‘Path of raw data is ’ + self.raw_data_path + ‘/’ + ‘’
def __len__(self):
return len(self.SampleInfoList)
def __getitem__(self, ndx):
SampleInfoList_tup = self.SampleInfoList[ndx]
filepath = self.raw_data_path + ‘/’ + str(SampleInfoList_tup.SampleName)
if os.path.exists(filepath):
img = Image.open(filepath)
# PIL image preprocess (examples)
#resize
if self.resize:
width, height = img.size
if (width 》= height) & (self.newsize[0] 》= self.newsize[1]):
img = pil_transform.resize(img, width=self.newsize[0], height=self.newsize[1])
elif (width 》= height) & (self.newsize[0] 《 self.newsize[1]):
img = pil_transform.resize(img, width=self.newsize[1], height=self.newsize[0])
elif (width 《 height) & (self.newsize[0] 《= self.newsize[1]):
img = pil_transform.resize(img, width=self.newsize[0], height=self.newsize[1])
elif (width 《 height) & (self.newsize[0] 》 self.newsize[1]):
img = pil_transform.resize(img, width=self.newsize[1], height=self.newsize[0])
else:
print(“ERROR”)
# from pil image to tensor
img_t = to_tensor(img)
# tensor preprocess (examples)
#rotation
ratio = img_t.shape[1]/img_t.shape[2]
if ratio 》 1:
img_t = torch.rot90(img_t, 1, [1, 2])
#normalization requires the knowledge of all tensors
if self.norm:
img_t = normalize(img_t)
#return img_t, SampleInfoList_tup
return img_t, SampleInfoList_tup.SampleLabel
else:
print(‘[WARNING] file {} does not exist’.format(str(SampleInfoList_tup.SampleName)))
return None
審核編輯:郭婷
-
機器學習
+關(guān)注
關(guān)注
66文章
8421瀏覽量
132703 -
python
+關(guān)注
關(guān)注
56文章
4797瀏覽量
84739 -
pytorch
+關(guān)注
關(guān)注
2文章
808瀏覽量
13236
發(fā)布評論請先 登錄
相關(guān)推薦
利用Arm Kleidi技術(shù)實現(xiàn)PyTorch優(yōu)化
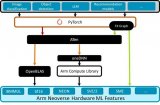
評論