FasterTransformer GPT 介紹(翻譯)
GPT 是 Decooding 模型的一種變體,沒有 Encoder 模塊,沒有交叉多頭注意力模塊,使用 GeLU 作為激活函數(shù)。2020 年,OpenAI 在他們的論文中表明,使用非常龐大的模型和大量的訓(xùn)練數(shù)據(jù)可以顯著提高 GPT 模型的容量。但是,不可能將這樣的模型放入單個 GPU 中。例如,最大的模型 GPT-3 有 1750 億個參數(shù),half 數(shù)據(jù)類型下大約需要 350 GB顯存。因此,多GPU,甚至多節(jié)點,是很有必要的。為了解決模型大小導(dǎo)致的延遲和內(nèi)存瓶頸,F(xiàn)asterTransformer 提供了高性能、低內(nèi)存占用的 kernel,并使用了模型并行技術(shù)。
支持的特性
Checkpoint converter
Huggingface
Megatron
Nemo Megatron
Data type
限制:
Note:
權(quán)重被切分后,隱藏層的維度必須是 64 的倍數(shù)。
cuda kernel通常只為小的 batch(如32和64)和權(quán)重矩陣很大時提供性能優(yōu)勢。
權(quán)重的 PTQ 量化只支持 FP16/BF16。
僅支持 Volta 和更新的 GPU 架構(gòu)。
根據(jù)當前 GPU 的情況,權(quán)重被提前離線預(yù)處理,以降低 TensorCore 做權(quán)重對齊的開銷。目前,我們直接使用 FP32/BF16/FP16 權(quán)重并在推理前對其進行量化。如果我們想存儲量化的權(quán)重,必須要在推理的 GPU 上來進行預(yù)處理。
使用 torch API 時,int8 模式只能通過 Parallel GPT Op 使用。Parallel GPT Op 也可以在單個 GPU 上使用。
FP32
FP16
BF16
INT8 weight only PTQ.
INT8 with SmoothQuant
FP8 (Experimental)
Feature
Multi-GPU multi-node inference
Dynamic random seed
Stop tokens
Beam search and sampling are both supported
Loading FP32 or FP16 weights
Frameworks
TensorFlow
PyTorch
Triton backend
FasterTransformer GPT 結(jié)構(gòu)(翻譯)
工作流
Fig 1. Workflow of GPT model
Fig 1展示了 FasterTransformer GPT 的工作流程。與 BERT 和編碼器-解碼器結(jié)構(gòu)不同,GPT 接收一些輸入 id 作為上下文,并生成相應(yīng)的輸出 id 作為響應(yīng)。在這個工作流中,主要的瓶頸是 GptDecoderLayer (Transformer塊),因為當我們增加層數(shù)的時候耗時也是線性增加的。在GPT-3中,GptDecoderLayer占用了大約95%的時間。
FasterTransformer把整個工作流分成了兩個部分。第一個部分是:“根據(jù)上下文context(也就是輸入ids)計算k/v cache”。第二個部分是:“自回歸的生成輸出ids”。這兩部分的操作類似,但是selfAttention部分的輸入tensors的形狀是不一樣的。所以FasterTransformer提供了2種計算方式,如Fig2所示。
在DecoderSelfAttention里面,query的序列長度總是1,所以我們使用自定義的fused masked multi-head attention kernel 進行處理。另一方面,在ContextSelfAttention中,query的序列長度最大時輸入的長度,所以我們使用cuBLAS來利用TensorCore。
Fig 2. Comparison between different self attention.
這個地方?jīng)]有理解為什么要分成2個Attention,因為自回歸的解碼也是需要把輸入的句子 padding 到最大的長度吧。這里的seq_len為1的情況是什么時候發(fā)生呢?我看了一下hugging face的GPT,似乎沒有找到對應(yīng)的位置。然后在FasterTransformer的GPT C++實現(xiàn)中也沒有找到這個DecoderSelfAttention的實現(xiàn):https://github.com/NVIDIA/FasterTransformer/blob/main/src/fastertransformer/models/multi_gpu_gpt/ParallelGptContextDecoder.cc 。不過本文主要是在后面介紹下 FasterTransformer 的優(yōu)化點以及優(yōu)缺點,這個暫時不影響解讀。
以下示例演示如何運行多 GPU 和多節(jié)點 GPT 模型。
examples/cpp/multi_gpu_gpt_example.cc: 它使用 MPI 來組織所有 GPU。
examples/cpp/multi_gpu_gpt_triton_example.cc: 它在節(jié)點內(nèi)使用多線程,節(jié)點間使用 MPI。此示例還演示了如何使用 FasterTransformer 的 Triton 后端 API 來運行 GPT 模型。
examples/pytorch/gpt/multi_gpu_gpt_example.py: 這個例子和 examples/cpp/multi_gpu_gpt_example.cc 很類似, 但是通過 PyTorch OP 封裝了 FasterTransformer 的實例。
總之,運行 GPT 模型的工作流程是:
通過 MPI 或多線程初始化 NCCL 通信并設(shè)置張量并行和流水并行的等級。
按張量并行、流水并行的ranks和其它模型超參數(shù)加載權(quán)重。
按張量并行,流水并行的ranks和其它模型超參數(shù)創(chuàng)建ParalelGpt實例。
接收來自客戶端的請求并將請求轉(zhuǎn)換為ParallelGpt的輸入張量格式.
運行 forward 函數(shù)
將 ParallelGpt 的輸出張量轉(zhuǎn)換為客戶端的響應(yīng)并返回響應(yīng)。
在c++示例代碼中,我們跳過第4步和第6步,通過examples/cpp/multi_gpu_gpt/start_ids.csv加載請求。在 PyTorch 示例代碼中,請求來自 PyTorch 端。在 Triton 示例代碼中,我們有從步驟 1 到步驟 6 的完整示例。
源代碼放在 src/fastertransformer/models/multi_gpu_gpt/ParallelGpt.cc 中。GPT的參數(shù)、輸入張量和輸出張量:
Constructor of GPT
Classification | Name | Data Type | Description |
---|---|---|---|
[0] | max_batch_size | size_t | Deprecated, move to input |
[1] | max_seq_len | size_t | Deprecated, move to input |
[2] | max_input_len | size_t | Deprecated, move to input |
[3] | beam_width | size_t | Deprecated, move to input |
[4] | head_num | size_t | Head number for model configuration |
[5] | size_per_head | size_t | Size per head for model configuration |
[6] | inter_size | size_t | The inter size of feed forward network. It is often set to 4 * head_num * size_per_head. |
[7] | num_layer | size_t | Number of transformer layers for model configuration |
[8] | vocab_size | int | Vocabulary size for model configuration |
[9] | start_id | int | Start id for vocabulary |
[18] | temperature | float | Deprecated, move to input |
[19] | len_penalty | float | Deprecated, move to input |
[20] | repetition_penalty | float | Deprecated, move to input |
[21] | tensor_para | NcclParam | Tensor Parallel information, which is declared in src/fastertransformer/utils/nccl_utils.h |
[22] | pipeline_para | NcclParam | Pipeline Parallel information, which is declared in src/fastertransformer/utils/nccl_utils.h |
[23] | stream | cudaStream_t | CUDA stream |
[24] | cublas_wrapper | cublasMMWrapper* | Pointer of cuBLAS wrapper, which is declared in src/fastertransformer/utils/cublasMMWrapper.h |
[26] | is_free_buffer_after_forward | bool | 如果設(shè)置為 true,F(xiàn)asterTransformer 將在 forward 前分配緩沖區(qū),并在 forward 后釋放緩沖區(qū)。當分配器基于內(nèi)存池時,設(shè)置為“true”可能有助于減少推理期間的內(nèi)存使用。 |
[27] | cuda_device_prop | cudaDeviceProp* | CUDA 設(shè)備屬性指針,用于獲取共享內(nèi)存大小等硬件屬性 |
[28] | sparse | bool | Is using sparsity. Experimental feature |
[29] | int8_mode | int | 0 means no quantization. 1 means use weight-only PTQ Experimental feature. 2 for weight and activation quantization Experimental feature. |
[30] | custom_all_reduce_comm | AbstractCustomComm | Custom all reduction communication for custom all reduction in model parallelism. It is only supported in 8-way tensor parallelism |
[31] | enable_custom_all_reduce | int | Flag of enabling custom all reduction or not |
[32] | remove_padding | bool | Remove the padding of input ids or not in context phase. |
[33] | shared_contexts_ratio | float | 控制共享上下文優(yōu)化使用的比率。If the compact size (that accounts only for unique prompts) is less than ratio * batch size,使用優(yōu)化的實現(xiàn) 。設(shè)置 shared_contexts_ratio=0 停用優(yōu)化。 |
Input of GPT
Name | Tensor/Parameter Shape | Location | Data Type | Description |
---|---|---|---|---|
input_ids | [batch_size, max_input_length] | GPU | int | The input ids (context) |
input_lengths | [batch_size] | GPU | int | The lengths of input ids |
prompt_learning_task_name_ids | [batch_size] | CPU | int | Optional. Task name ids for prompt learning. |
output_seq_len | [batch_size] | CPU | uint32_t | The largest number of tokens you hope for results. Note that it contains the input length |
stop_words_list | [batch_size, 2, stop_words_length] | GPU | int | Optional. When FT generates words in this list, it will stop the generation. An extension of stop id |
bad_words_list | [batch_size, 2, bad_words_length] | GPU | int | Optional. The words in the list will never be sampled. |
repetition_penalty | [1] or [batch_size] | CPU | float | Optional. Repetition penalty applied to logits for both beam search and sampling. Exclusive with presence_penalty. |
presence_penalty | [1] or [batch_size] | CPU | float | Optional. Presence penalty - additive type of repetition penalty - applied to logits for both beam search and sampling. Exclusive with repetition_penalty. |
min_length | [1] or [batch_size] | CPU | int | Optional. Minimum number of tokens to generate |
random_seed | [1] or [batch_size] | CPU | unsigned long long int | Optional. Random seed to initialize the random table in sampling. |
request_prompt_lengths | [batch_size], | GPU | int | Optional. Length of prefix soft prompt embedding. This describes how many tokens of soft prompt embedding in each sentence. |
request_prompt_embedding | [batch_size, max_prompt_length, hidden_units] | GPU | float/half/bfloat16 | Optional. FT will concat them with results of embedding lookup kernel. For prefix soft prompt embedding, the type must be float; for p/prompt tuning, the type is same to weight. |
request_prompt_type | [batch_size] | CPU | int | Optional. Prompt type of request. This is necessary when user pass the prompt embedding by input |
is_return_context_cum_log_probs | [1] | CPU | bool | Optional. Return the cumulative log probability of context or not |
is_return_context_embeddings | [1] | CPU | bool | Optional. Return the sum of context tokens encodings or not |
session_len | [1] | CPU | uint32 | Optional. The maximum time length allowed during the whole interactive generation. Only used for interactive generation feature |
continue_gen | [1] | CPU | bool | Optional. A flag to tell FasterTransformer to not discard previous tokens and continue producing token based on previous generations. Only used for interactive generation feature |
memory_len | [1] | CPU | uint32 | Optional. The maximum time memory used in attention modules. Reduces the memory footprint but quality of generation might degrades. |
top_p_decay | [batch_size] | GPU | float | Optional. decay values for top_p sampling |
top_p_min | [batch_size] | GPU | float | Optional. min top_p values for top p sampling |
top_p_reset_ids | [batch_size] | GPU | uint32 | Optional. reset ids for resetting top_p values for top p sampling |
Output of GPT
Name | Tensor/Parameter Shape | Location | Data Type | Description |
---|---|---|---|---|
output_ids | [batch_size, beam_width, max_output_seq_len] | GPU | int | The output ids. It contains the input_ids and generated ids |
sequence_length | [batch_size, beam_width] | GPU | int | The lengths of output ids |
output_log_probs | [batch_size, beam_width, request_output_seq_len] | GPU | float | Optional. It records the log probability of logits at each step for sampling. |
cum_log_probs | [batch_size, beam_width] | GPU | float | Optional. Cumulative log probability of generated sentences |
context_embeddings | [batch_size, beam_width, hidden_units] | GPU | float | Optional. Sum of context tokens encodings. |
beam_width 值直接由輸出形狀設(shè)置。當output_ids的beam_width大于1時,F(xiàn)T會使用beam search來生成token;否則,F(xiàn)T 將使用 topk 或 topp 采樣。當 beam search 和 sampling 的輸入無效時,比如 beam width 1,top k 0,top p 0.0,F(xiàn)T 會自動運行 greedy search。·
優(yōu)化
kernel 優(yōu)化:很多 kernel 都是基于已經(jīng)高度優(yōu)化的解碼器和解碼模塊的 kernel。為了防止重新計算以前的key 和 value,我們將在每一步分配一個緩沖區(qū)來存儲它們。雖然它需要一些額外的內(nèi)存使用,但我們可以節(jié)省重新計算的成本,在每一步分配緩沖區(qū)以及串行的成本。
內(nèi)存優(yōu)化:與 BERT 等傳統(tǒng)模型不同,GPT-3 有 1750 億個參數(shù),即使我們以半精度存儲模型也需要 350 GB。因此,我們必須減少其他部分的內(nèi)存使用。在 FasterTransformer 中,我們將重用不同解碼器層的內(nèi)存緩沖區(qū)。由于 GPT-3 的層數(shù)是 96,我們只需要 1/96 的內(nèi)存。
模型并行:在GPT模型中,F(xiàn)asterTransormer同時提供張量并行和流水線并行。對于張量并行,F(xiàn)asterTransformer 遵循了 Megatron 的思想。對于自注意力塊和前饋網(wǎng)絡(luò)塊,我們按行拆分第一個矩陣乘法的權(quán)重,按列拆分第二個矩陣乘法的權(quán)重。通過優(yōu)化,我們可以將每個Transformer塊的歸約操作減少到 2 次。工作流程如Fig 3 所示。對于流水并行,F(xiàn)asterTransformer 將整個Batch的請求拆分為多個Micro Batch并隱藏通信氣泡。FasterTransformer 會針對不同情況自動調(diào)整微批量大小。用戶可以通過修改 gpt_config.ini 文件來調(diào)整模型并行度。我們建議在節(jié)點內(nèi)使用張量并行,在節(jié)點間使用流水并行,因為張量并行需要更多的 NCCL 通信。
多框架:FasterTransformer除了C上的源代碼,還提供了TensorFlow op、PyTorch op和Triton backend。目前TensorFlow op只支持單GPU,而PyTorch op和Triton backend支持多GPU和多節(jié)點。FasterTransformer 還提供了一個工具,可以將 Megatron 的模型拆分并轉(zhuǎn)換為二進制文件,然后 FasterTransformer 可以直接加載二進制文件,從而避免為模型并行而進行額外的模型拆分工作。
Fig 3. Workflow of GPT with tensor parallelism.
筆記
is_context_qk_buf_float_(是否對 GPT context QK GEMM 使用浮點累加)默認設(shè)置為 false。如果您遇到與 GPT Context注意力模塊相關(guān)的準確性問題,請嘗試在 ParallelGpt.h 中啟用它。
CUDA相關(guān)優(yōu)化點解讀
TensorRT fused multi-head attention kernel: 和 BERT 一樣對于 GPT 的 ContextSelfAttention,F(xiàn)asterTransformer 使用 TensorRT 的 fused multi-head attention kernel 將 batch GEMM,softmax, GEMM,transpose 等操作都合并成一個 cuda kernel,不僅可以減少數(shù)據(jù)搬提升帶寬利用率還可以減少 kernel launch 的開銷。
AddBiasResidualLayerNorm:在 Decoder 中將 Attention 的最后一個 Linear 的 bias_add,殘差連接(elementwise_add)以及 LayerNorm 合并成一個 AddBiasResidualLayerNorm Kernel,降低 Kernel Launch 開銷以及提升訪問帶寬。
GeluFFN:從Fig1中的示意圖可以看到 GeluFFN 包含兩個 Linear 層,中間夾了一個 GeLU 的激活函數(shù),這里做的優(yōu)化是把第一個 Linear 層的 bias_add 和 GeLU 激活函數(shù) fuse 到一起,也就是 AddBiasGeLU Kernel。
AddBiasResidual:從Fig1的示意圖可以看到,Decoder的最后一層就是 AddBiasResidual,這個Kernel就是把 bias_add 和 殘差連接(element_wise add) 融合到一起。
GEMM 試跑:和BERT一樣仍然是在運行模型之前先試跑一下 GPT 網(wǎng)絡(luò)中涉及到的GEMM的尺寸,并且保存 GEMM 性能最高的超參數(shù)配置,這個對于 cublas 和 cutlass 實現(xiàn)的卷積應(yīng)該都是成立的。
高效的 LayerNorm:在 TensorFlow 里面 LayerNorm 是零碎的 Kernel 拼接的,在 FasterTransformer 中實現(xiàn)了一個 LayerNorm Kernel 來完成這個功能。實際上 PyTorch/OneFlow 等框架也有 LayerNorm Kernel,并且 OneFlow 的 LayerNorm 性能最強。
GEMM 的 FP16 累加:上面提到 is_context_qk_buf_float_ 參數(shù),在 GPT 的 fp8 實現(xiàn)中,默認使用 GEMM 的 FP16 累加而非 FP32 累加,進一步提升性能,但是也可能帶來精度問題。
最近我做一個大模型的推理工作時也發(fā)現(xiàn)如果基于 cutlass 的 gemm 使用 FP16 累加,最后生成的結(jié)果會部分亂碼,所以這個優(yōu)化必須用環(huán)境變量或者類似于這里用一個單獨的參數(shù)來控制。
和通信相關(guān)的實現(xiàn)以及shared_context相關(guān)的優(yōu)化這里就不提了,代碼的可讀性比較差,我個人建議有需要的讀者學(xué)習(xí)下某些kernel的實現(xiàn)即可。
FasterTransformer 優(yōu)點
從之前對 BERT 的優(yōu)化點介紹以及這里對 GPT 的優(yōu)化點介紹,我們可以發(fā)現(xiàn)FasterTransformer集成了大量針對Transformer架構(gòu)的優(yōu)化,并且實現(xiàn)了各種Transformer架構(gòu)中常見的各種fuse pattern對應(yīng)的kernel。并且較為完整的支持了Transformer架構(gòu)的int8推理,整體的性能始終保持在一個SOTA水平。對于我這種入門CUDA優(yōu)化的學(xué)習(xí)者來說有一定的學(xué)習(xí)意義。此外,F(xiàn)asterTransformer也將實現(xiàn)的這些組件注冊到TensorFlow,PyTorch等框架中使得讀者可以對常見的Transformer架構(gòu)進行推理。
FasterTransformer 缺點
CUDA Kernel之外的代碼寫得很抽象,特別對于多卡模式來說需要用戶手動管理通信和模型切分,這個門檻是很高的。如果用戶想基于FasterTreansformer這個框架實現(xiàn)新的Transformer架構(gòu)的網(wǎng)絡(luò)會非常困難,必須要非常了解FasterTransformer才可以。除了要手動管理通信以及模型切分之外,如果用戶的新模型中出現(xiàn)了新的組件不僅要實現(xiàn)CUDA Kernel還需要手動管理內(nèi)存的申請和釋放,比如GPT的內(nèi)存申請和釋放:
最近試跑了一個第三方模型的FasterTransformer實現(xiàn),就出現(xiàn)了類似的問題。
個人認為 FasterTransformer 的整體架構(gòu)實現(xiàn)的用戶體驗類似于 “九轉(zhuǎn)大腸”,易用性方面我還是比較看好 PyTorch ,OneFlow 等將內(nèi)存管理,通信集成到框架底層用戶新增模型只需要關(guān)心自定義 CUDA Kernel 的傳統(tǒng)深度學(xué)習(xí)框架。個人建議可以學(xué)習(xí)下 FasterTransformer 某些 CUDA Kernel 實現(xiàn),但基于這個框架來搭建應(yīng)用要慎重。如果基于 PyTorch,OneFlow 等框架能將大量的 Transformer 架構(gòu)性能追平甚至超越 FasterTransformer 就完全沒必要折磨自己。
總結(jié)
這里總結(jié)了一下 FasterTransformer 里面和 CUDA Kernel相關(guān)的優(yōu)化技巧,并且給出了Kernel實現(xiàn)的位置,并從易用性,性能多方便對比了 FasterTransformer 和 PyTorch/OneFlow 等框架的優(yōu)缺點,供大家參考學(xué)習(xí)。
審核編輯:劉清
-
編碼器
+關(guān)注
關(guān)注
45文章
3663瀏覽量
135027 -
gpu
+關(guān)注
關(guān)注
28文章
4766瀏覽量
129198 -
GPT
+關(guān)注
關(guān)注
0文章
357瀏覽量
15461 -
API串口
+關(guān)注
關(guān)注
0文章
13瀏覽量
4859
原文標題:【BBuf的CUDA筆記】七,總結(jié) FasterTransformer Decoder(GPT) 的cuda相關(guān)優(yōu)化技巧
文章出處:【微信號:GiantPandaCV,微信公眾號:GiantPandaCV】歡迎添加關(guān)注!文章轉(zhuǎn)載請注明出處。
發(fā)布評論請先 登錄
相關(guān)推薦
使用TC21x的GPT實現(xiàn)1m計時器執(zhí)行定時任務(wù),怎么配置GTM和GPT?
GPT定時器?基本知識詳解
EPIT定時器與GPT定時器簡單介紹
GPT高精度延時定時器簡介
GPT2模塊的相關(guān)資料推薦
瑞芯微在開源支持中使用GPT作為其主要分區(qū)表
IMX6ULL中如何使用GPT2的capture1捕捉外部PWM信號?
UEFI+GPT、Legacy+MBR引導(dǎo)模式介紹及引導(dǎo)修復(fù)教程
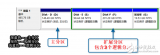
專家解讀GPT 2.0 VS BERT!GPT 2.0到底做了什么
NVIDIA FasterTransformer庫的概述及好處
使用FasterTransformer和Triton推理服務(wù)器部署GPT-J和T5
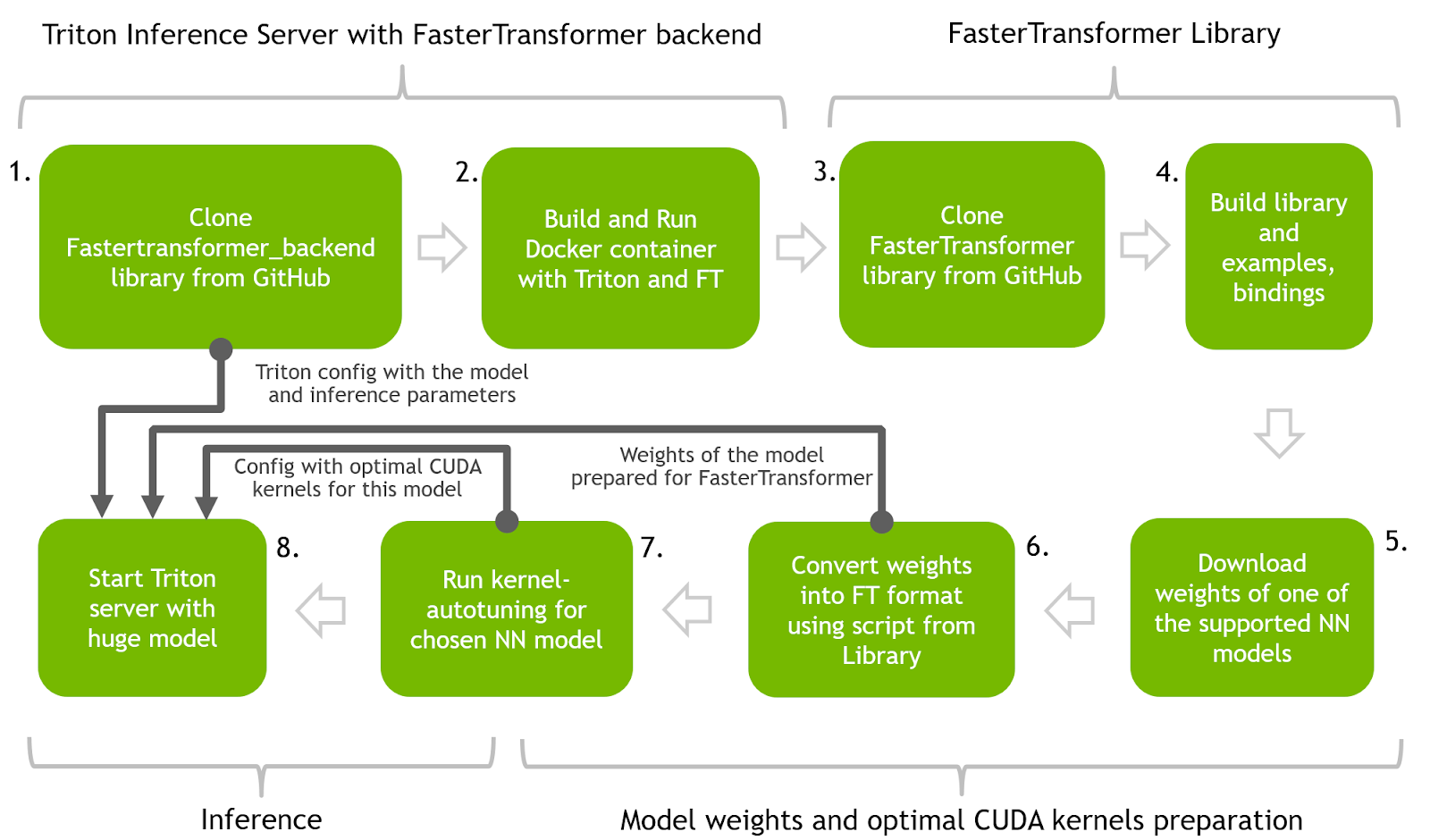
總結(jié)FasterTransformer Encoder(BERT)的cuda相關(guān)優(yōu)化技巧
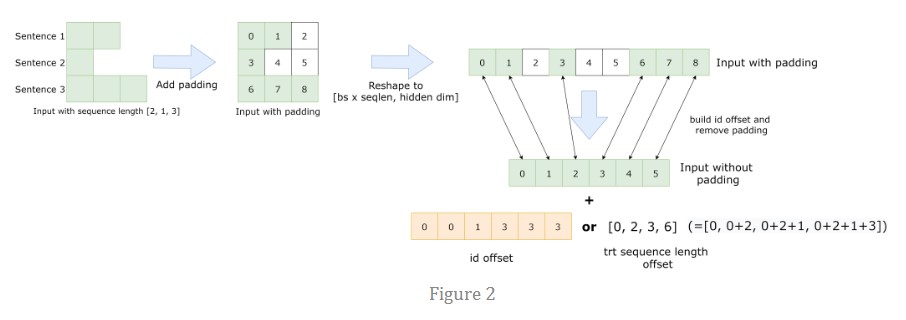
GPT/GPT-2/GPT-3/InstructGPT進化之路
總結(jié)FasterTransformer Encoder優(yōu)化技巧
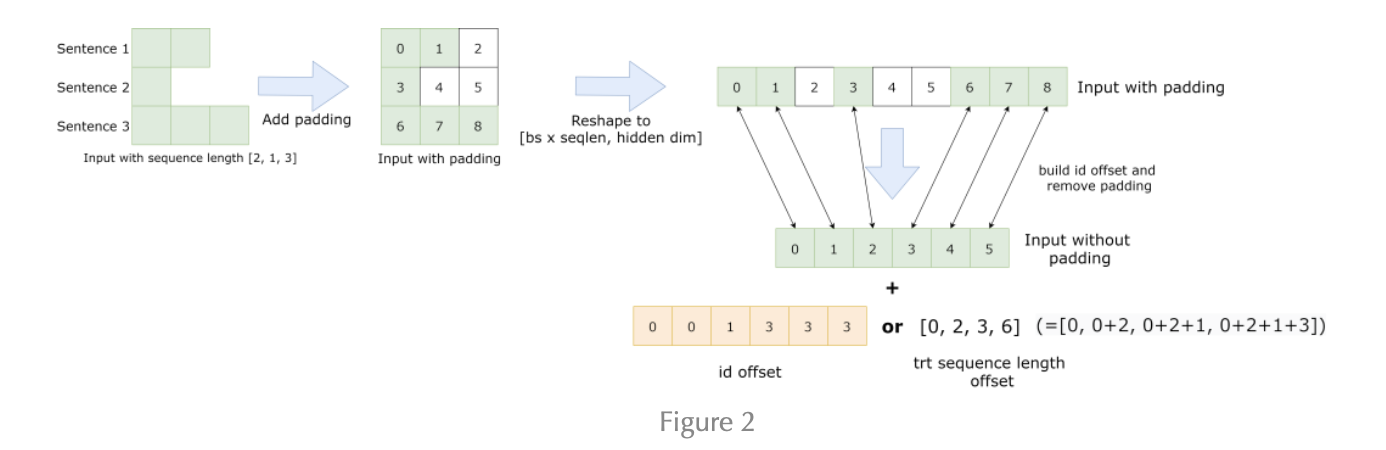
評論