ESIM是ACL2017的一篇論文,在當(dāng)時成為各個NLP比賽的殺器,直到現(xiàn)在仍是入門自然語言推理值得一讀的文章。
本文根據(jù)ESIM原文以及pytorch代碼實(shí)現(xiàn)對ESIM模型進(jìn)行總結(jié),有些地方的敘述保持了與代碼一致而和原文不一致,比如在embedding處與原文就不完全一致,原論文只使用了我下面所寫的initial embedding,不過在代碼性能上應(yīng)該是不會比原文的更差的,因?yàn)榇a過長,僅放一些偽代碼幫助理解。計(jì)算過程公式稍多,但無非是LSTM和Attention,理解起來并不太困難。
介紹 Introduction
自然語言推斷 NLI
NLI任務(wù)主要是關(guān)于給定前提premise和假設(shè)hypothesis,要求判斷p和h的關(guān)系,二者的關(guān)系有三種:1.不相干 neural,2.沖突 contradiction,即p和h有矛盾,3.蘊(yùn)含 entailment,即能從p推斷出h或兩者表達(dá)的是一個意思。
?為什么要研究自然語言推理呢?簡單來講,機(jī)器學(xué)習(xí)的整個系統(tǒng)可以分為兩塊,輸入,輸出。輸入要求我們能夠輸入一個機(jī)器能理解的東西,并且能夠很好的表現(xiàn)出數(shù)據(jù)的特點(diǎn),輸出就是根據(jù)需要,生成我們需要的結(jié)果。也可以說整個機(jī)器學(xué)習(xí)可以分為Input Representation和Output Generation。因此,如何全面的表示輸入就變得非常重要了。而自然語言推理是一個分類任務(wù),使用準(zhǔn)確率就可以客觀有效的評價模型的好壞;這樣我們就可以專注于語義理解和語義表示。并且如果這部分做得好的話,例如可以生成很好的句子表示的向量,那么我們就可以將這部分成果輕易遷移到其他任務(wù)中,例如對話,問答等。這一切都說明了研究自然語言推理是一個非常重要而且非常有意義的事情。
?
下面從Stanford Natural Language Inference (SNLI) corpus數(shù)據(jù)集里舉幾個例子:
A woman with a green headscarf , blue shirt and a very big grin(咧嘴笑).
The woman is very happy .
上面兩個句子就是 「entailment(蘊(yùn)含)」 ,因?yàn)榕嗽谛χ?,所以說她happy是可以推斷出來的。
A woman with a green headscarf , blue shirt and a very big grin .
The woman is young .
「neutral」
沖突矛盾(contradiction)的例子
A woman with a green headscarf , blue shirt and a very big grin.
The woman has been shot .
「contradiction」
她中槍了怎么可能還咧嘴笑呢?
模型架構(gòu) Models
輸入編碼 Input Encoding
輸入兩個句子,從one-hot經(jīng)過embedding層,有兩個embedding層,分別是initial embedding( 「ie」 ) 和 pretrained embedding( 「pe」 ),都使用預(yù)訓(xùn)練好的詞向量初始化,詞向量維度為,不同的是 ie 的詞表規(guī)模是訓(xùn)練集語料的單詞個數(shù),pe 的詞表規(guī)模就是預(yù)訓(xùn)練文件所包含的單詞數(shù),且 pe 參數(shù)被凍結(jié),ie中沒被包含在預(yù)訓(xùn)練文件的OOV單詞使用高斯分布隨機(jī)生成,且所有embedding的方差都被normalize到1,得到和,每個單詞的表示是一個 的向量,由其在 ie 和 pe 中對應(yīng)的詞向量 concat 得到, 為預(yù)訓(xùn)練詞向量維度,
src_words, src_extwords_embed, src_lens, src_masks,
tgt_words, tgt_extwords_embed, tgt_lens, tgt_masks = tinputs
src_dyn_embed = self.word_embed(src_words)
tgt_dyn_embed = self.word_embed(tgt_words)
src_embed = torch.cat([src_dyn_embed, src_extwords_embed], dim=-1)
tgt_embed = torch.cat([tgt_dyn_embed, tgt_extwords_embed], dim=-1)
之后使用雙向LSTM分別對a和b進(jìn)行encoding,得到兩個句子的隱層狀態(tài)表示,論文中隱層向量的維度等于預(yù)訓(xùn)練詞向量的維度,因?yàn)槭莃idirectional = True,所以 。
src_hiddens = self.lstm_enc(src_embed, src_lens)
tgt_hiddens = self.lstm_enc(tgt_embed, tgt_lens)
局部推理 Locality of inference
就是使用attention建立p和h之間的聯(lián)系,即進(jìn)行對齊操作,a和b中兩個單詞的注意力權(quán)重由向量內(nèi)積得到。
Local inference collected over sequences(不知道咋翻譯)
接著利用得到的注意力權(quán)重,對b進(jìn)行加權(quán)求和,即從b中選取與相關(guān)的部分來得到表示,對b同理
similarity_matrix = premise_batch.bmm(hypothesis_batch.transpose(2, 1).contiguous())
# hyp_mask shape = [batch_size, tgt_len]
prem_hyp_attn = masked_softmax(similarity_matrix, hypothesis_mask)
# prem_mask shape = [batch_size, src_len]
hyp_prem_attn = masked_softmax(similarity_matrix.transpose(1, 2).contiguous(), premise_mask)
# Weighted sums of the hypotheses for the the premises attention,
# [batch_size, src_len, hidden_size]
src_hiddens_att = weighted_sum(hypothesis_batch,
prem_hyp_attn,
premise_mask)
# [batch_size, tgt_len, hidden_size]
tgt_hiddens_att = weighted_sum(premise_batch,
hyp_prem_attn,
hypothesis_mask)
局部推理信息增強(qiáng) Enhancement of local inference information
現(xiàn)在a的每個單詞有兩個vector表示,分別是和,b亦然,再對兩個vector分別做element-wise的減法與乘法,并把它們 concat 到一起,得到維度為原來四倍長的vector,
src_diff_hiddens = src_hiddens - src_hiddens_att
src_prod_hiddens = src_hiddens * src_hiddens_att
# [batch_size, src_len, 2 * lstm_hiddens * 4] 乘2是雙向
src_summary_hiddens = torch.cat([src_hiddens, src_hiddens_att, src_diff_hiddens,
src_prod_hiddens], dim=-1)
tgt_diff_hiddens = tgt_hiddens - tgt_hiddens_att
tgt_prod_hiddens = tgt_hiddens * tgt_hiddens_att
tgt_summary_hiddens = torch.cat([tgt_hiddens, tgt_hiddens_att, tgt_diff_hiddens,
tgt_prod_hiddens], dim=-1)
推理合成 Inference Composition
繼續(xù)使用LSTM提取特征,得到兩個句子因果關(guān)系表示。因?yàn)?concat 操作會使得參數(shù)量數(shù)倍增長,為了防止參數(shù)過多導(dǎo)致的過擬合,把和經(jīng)過一個激活函數(shù)為ReLU的全連接層,將維度從投影到,這樣之后再經(jīng)過一個BiLSTM層,得到
src_hiddens_proj = self.mlp(src_summary_hiddens)
tgt_hiddens_proj = self.mlp(tgt_summary_hiddens)
# [batch_size, src_len, 2 * lstm_hiddens]
src_final_hiddens = self.lstm_dec(src_hiddens_proj, src_lens)
tgt_final_hiddens = self.lstm_dec(tgt_hiddens_proj, tgt_lens)
池化層 Pooling
將組成整句話的sequence vectors分別通過 average pooling 和 max pooling(element-wise),變成單獨(dú)的一個vector,并將它們再次 concat 起來,得到能完整表示p和h以及兩者之間關(guān)系的final向量v
最后將他們送入分類層,分類層包括兩個全連接層,中間是tanh激活函數(shù),輸出維度為標(biāo)簽種類個數(shù)。
hiddens = torch.cat([src_hidden_avg, src_hidden_max, tgt_hidden_avg, tgt_hidden_max], dim=1)
# [batch_size, tag_size]
outputs = self.proj(hiddens)
實(shí)驗(yàn) Experiments
數(shù)據(jù)集 Data
數(shù)據(jù)集使用的是Stanford Natural Language Inference (SNLI) corpus,每條數(shù)據(jù)是三個句子,分別代表premise, hypothesis和tag
訓(xùn)練參數(shù)設(shè)置 Training
使用Adam優(yōu)化函數(shù),lr=0.0004,batch_size=32,所有LSTM的隱層狀態(tài)維度皆為300,dropout也被在各個層中使用且p=0.5,預(yù)訓(xùn)練詞向量使用的是glove.840B.300d,在SNLI數(shù)據(jù)集上達(dá)到了88%的acc。
實(shí)驗(yàn)結(jié)果
HIM是使用Tree-LSTM引入了句法信息的方法,較為復(fù)雜不再贅述,有興趣的同學(xué)可以去閱讀原文。
-
eSIM
+關(guān)注
關(guān)注
3文章
241瀏覽量
26612 -
自然語言
+關(guān)注
關(guān)注
1文章
288瀏覽量
13350 -
nlp
+關(guān)注
關(guān)注
1文章
488瀏覽量
22037 -
pytorch
+關(guān)注
關(guān)注
2文章
808瀏覽量
13226
發(fā)布評論請先 登錄
相關(guān)推薦
python自然語言
自然語言處理怎么最快入門?
【推薦體驗(yàn)】騰訊云自然語言處理
自然語言處理怎么最快入門_自然語言處理知識了解
自然語言處理入門基礎(chǔ)之hanlp詳解
PyTorch教程16.4之自然語言推理和數(shù)據(jù)集
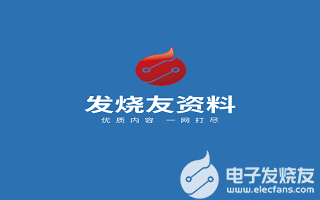
PyTorch教程16.5之自然語言推理:使用注意力
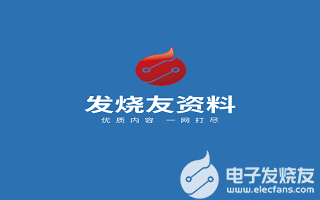
PyTorch教程16.7之自然語言推理:微調(diào)BERT
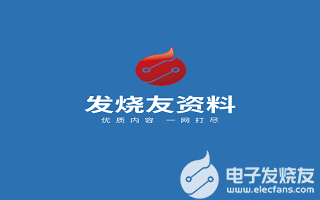
評論