【前言】 RT-DETR是由百度近期推出的DETR-liked目標(biāo)檢測(cè)器,該檢測(cè)器由HGNetv2、混合編碼器和帶有輔助預(yù)測(cè)頭的Transformer編碼器組成,整體結(jié)構(gòu)如下所示。
本文將采用RT-DETR兩種不同風(fēng)格的onnx格式,使用onnxruntime20行代碼,無(wú)需nms操作即可實(shí)現(xiàn)簡(jiǎn)易部署推理.
一、原生onnx+ort推理方式
使用以下命令抽取出模型配置文件和模型參數(shù)文件:
pythontools/export_model.py-cconfigs/rtdetr/rtdetr_hgnetv2_l_6x_coco.yml-oweights=https://bj.bcebos.com/v1/paddledet/models/rtdetr_hgnetv2_l_6x_coco.pdparamstrt=True--output_dir=output_inference
轉(zhuǎn)化模型為onnx形式:
paddle2onnx--model_dir=./output_inference/rtdetr_hgnetv2_l_6x_coco/--model_filenamemodel.pdmodel--params_filenamemodel.pdiparams--opset_version16--save_filertdetr_hgnetv2_l_6x_coco.onnx
抽取后的onnx可視化如下:
可以看到,除了圖像的輸入,還有另外兩個(gè)輸入頭,其中,im_shape指原輸入圖像的尺寸,scale_factor指靜態(tài)圖尺度/原輸入圖像尺度,其實(shí)就是縮放的系數(shù)。
我們將batch_size固定為1,裁減掉不需要使用到的算子:
python-mpaddle2onnx.optimize--input_modelrtdetr_hgnetv2_l_6x_coco.onnx--output_modelrtdetr_hgnetv2_l_6x_coco_sim.onnx--input_shape_dict"{'image':[1,3,640,640]}
使用簡(jiǎn)化后的onnx模型進(jìn)行推理:
importonnxruntimeasrt importcv2 importnumpyasnp sess=rt.InferenceSession("/home/aistudio/PaddleDetection/rtdetr_hgnetv2_l_6x_coco_sim.onnx") img=cv2.imread("../000283.jpg") org_img=img im_shape=np.array([[float(img.shape[0]),float(img.shape[1])]]).astype('float32') img=cv2.resize(img,(640,640)) scale_factor=np.array([[float(640/img.shape[0]),float(640/img.shape[1])]]).astype('float32') img=img.astype(np.float32)/255.0 input_img=np.transpose(img,[2,0,1]) image=input_img[np.newaxis,:,:,:] result=sess.run(["reshape2_83.tmp_0","tile_3.tmp_0"],{'im_shape':im_shape,'image':image,'scale_factor':scale_factor}) forvalueinresult[0]: ifvalue[1]>0.5: cv2.rectangle(org_img,(int(value[2]),int(value[3])),(int(value[4]),int(value[5])),(255,0,0),2) cv2.putText(org_img,str(int(value[0]))+":"+str(value[1]),(int(value[2]),int(value[3])),cv2.FONT_HERSHEY_SIMPLEX,0.5,(255,255,255),1) cv2.imwrite("../result.png",org_img)
推理結(jié)果:
二、野生onnx+ort推理方式
其實(shí)通過(guò)官方onnx模型的格式可以看出,官方已經(jīng)將所有后處理步驟寫(xiě)入到模型中,此時(shí)不需要額外添加后處理代碼,是一種比較省心的方式。
但對(duì)于有強(qiáng)迫癥的筆者而言,對(duì)于三個(gè)輸入頭的模型實(shí)在是看著別扭,因此我更偏向于下面的這種推理方式。
同樣是抽取官方模型,但此時(shí)我們將后處理的所有操作全部摘除,只保留原模型參數(shù):將模型的exclude_post_process設(shè)置為T(mén)rue,然后使用同樣的代碼進(jìn)行轉(zhuǎn)化:
pythontools/export_model.py-cconfigs/rtdetr/rtdetr_hgnetv2_l_6x_coco.yml-oweights=https://bj.bcebos.com/v1/paddledet/models/rtdetr_hgnetv2_l_6x_coco.pdparamstrt=True--output_dir=output_inference_sim
將轉(zhuǎn)化后的pdmodel進(jìn)行可視化:
左邊為未摘除后處理的pdmodel,右邊為摘除后的pdmodel,以分類(lèi)支路為例,我們可以看到,分類(lèi)支路從Sigmoid開(kāi)始,已經(jīng)Sigmoid和后面的Children Node摘除干凈,那么可以轉(zhuǎn)化為onnx文件,步驟與上面一致。
使用轉(zhuǎn)化后的onnx文件進(jìn)行推理:
importonnxruntimeasrt importcv2 importnumpyasnp sess=rt.InferenceSession("rtdetr_hgnetv2_l_6x_coco_sim2.onnx") img=cv2.imread("../000283.jpg") img=cv2.resize(img,(640,640)) image=img.astype(np.float32)/255.0 input_img=np.transpose(image,[2,0,1]) image=input_img[np.newaxis,:,:,:] results=sess.run(['scores','boxes'],{'image':image}) scores,boxes=[o[0]foroinresults] index=scores.max(-1) boxes,scores=boxes[index>0.5]*640,scores[index>0.5] labels=scores.argmax(-1) scores=scores.max(-1) forbox,score,labelinzip(boxes,scores,labels): cx,cy,w,h=int(box[0]),int(box[1]),int(box[2]),int(box[3]) cv2.rectangle(img,(cx-int(w/2),cy-int(h/2)),(cx+int(w/2),cy+int(h/2)),(0,255,255),2) cv2.putText(img,f'{label}:{score:.2f}',(cx-int(w/2),cy-int(h/2)-5),cv2.FONT_HERSHEY_SIMPLEX,0.5,(0,255,255),1) cv2.imwrite('../result.jpg',img)
推理結(jié)果:
【結(jié)尾】
本文介紹了RT-DETR兩種風(fēng)格的onnx格式和推理方式,不管哪種風(fēng)格,精度無(wú)任何差別,至于是使用哪款,純憑個(gè)人愛(ài)好。
審核編輯:劉清
-
編碼器
+關(guān)注
關(guān)注
45文章
3643瀏覽量
134525 -
檢測(cè)器
+關(guān)注
關(guān)注
1文章
864瀏覽量
47688
原文標(biāo)題:無(wú)需nms,onnxruntime20行代碼玩轉(zhuǎn)RT-DETR
文章出處:【微信號(hào):GiantPandaCV,微信公眾號(hào):GiantPandaCV】歡迎添加關(guān)注!文章轉(zhuǎn)載請(qǐng)注明出處。
發(fā)布評(píng)論請(qǐng)先 登錄
相關(guān)推薦
vnc和xrdp兩種遠(yuǎn)程連接的方式
YOLOX模型ONNX格式說(shuō)明
在MATLAB/simulink中建模時(shí)的兩種不同實(shí)現(xiàn)方式
MATLAB/simulink中兩種實(shí)現(xiàn)建模方式的優(yōu)勢(shì)
簡(jiǎn)單聊聊目標(biāo)檢測(cè)新范式RT-DETR的骨干:HGNetv2
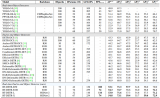
ONNX格式模型部署兼容性框架介紹
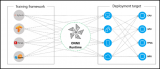
DETR架構(gòu)的內(nèi)部工作方式分析
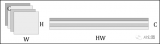
基于OpenVINO Python API部署RT-DETR模型
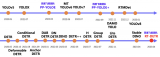
基于OpenVINO C++ API部署RT-DETR模型
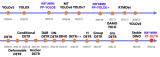
基于OpenVINO C# API部署RT-DETR模型
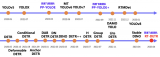
百度開(kāi)源DETRs在實(shí)時(shí)目標(biāo)檢測(cè)中勝過(guò)YOLOs
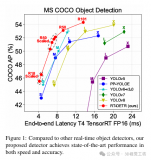
OpenVINO? Java API應(yīng)用RT-DETR做目標(biāo)檢測(cè)器實(shí)戰(zhàn)
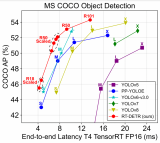
評(píng)論