前言
圖像分割可以分為兩類:語義分割(Semantic Segmentation)和實例分割(Instance Segmentation),前面已經給大家介紹過兩者的區別,并就如何在labview上實現相關模型的部署也給大家做了講解,今天和大家分享如何使用labview 實現deeplabv3+的語義分割,并就 Pascal VOC2012 (DeepLabv3Plus-MobileNet) 上的分割結果和城市景觀的分割結果(DeepLabv3Plus-MobileNet)給大家做一個分享。
一、什么是deeplabv3+
Deeplabv3+是一個語義分割網絡,使用DeepLabv3作為Encoder模塊,并添加一個簡單且有效的Decoder模塊來獲得更清晰的分割。即網絡主要分為兩個部分:Encoder和Decoder;論文中采用的是Xception作為主干網絡(在代碼中也可以根據需求替換成MobileNet,本文示例中即使用的MobileNet),然后使用了ASPP結構,解決多尺度問題;為了將底層特征與高層特征融合,提高分割邊界準確度,引入Decoder部分。
Encoder-Decoder網絡已經成功應用于許多計算機視覺任務,通常,Encoder-Decoder網絡包含:
- 逐步減少特征圖并提取更高語義信息的Encoder模塊
- 逐步恢復空間信息的Decoder模塊
二、LabVIEW調用DeepLabv3+實現圖像語義分割
1、模型獲取及轉換
-
下載預訓練好的.pth模型文件,下載鏈接:https://share.weiyun.com/qqx78Pv5 ,我們選擇主干網絡為Mobilenet的模型
-
git上下載開源的整個項目文件,鏈接為:https://github.com/VainF/DeepLabV3Plus-Pytorch
-
根據requirements.txt 安裝所需要的庫
pip install -r requirements.txt
- 原項目中使用的模型為.pth,我們將其轉onnx模型,
- 將best_deeplabv3plus_mobilenet_voc_os16.pth轉化為deeplabv3plus_mobilenet.onnx,具體轉化模型代碼如下:
import network
import numpy as np
import torch
from torch.autograd import Variable
from torchvision import models
import os
import re
dirname, filename = os.path.split(os.path.abspath(__file__))
print(dirname)
def get_pytorch_onnx_model(original_model):
# define the directory for further converted model save
onnx_model_path = dirname
# define the name of further converted model
onnx_model_name = "deeplabv3plus_mobilenet.onnx"
# create directory for further converted model
os.makedirs(onnx_model_path, exist_ok=True)
# get full path to the converted model
full_model_path = os.path.join(onnx_model_path, onnx_model_name)
# generate model input
generated_input = Variable(
torch.randn(1, 3, 513, 513)
)
# model export into ONNX format
torch.onnx.export(
original_model,
generated_input,
full_model_path,
verbose=True,
input_names=["input"],
output_names=["output"],
opset_version=11
)
return full_model_path
model = network.modeling.__dict__["deeplabv3plus_mobilenet"](num_classes=21, output_stride=8)
checkpoint = torch.load("best_deeplabv3plus_mobilenet_voc_os16.pth", map_location=torch.device('cpu'))
model.load_state_dict(checkpoint["model_state"])
full_model_path = get_pytorch_onnx_model(model)
- 將best_deeplabv3plus_mobilenet_cityscapes_os16.pth轉化為deeplabv3plus_mobilenet_cityscapes.onnx,具體轉化模型代碼如下:
import network
import numpy as np
import torch
from torch.autograd import Variable
from torchvision import models
import os
import re
dirname, filename = os.path.split(os.path.abspath(__file__))
print(dirname)
def get_pytorch_onnx_model(original_model):
# define the directory for further converted model save
onnx_model_path = dirname
# define the name of further converted model
onnx_model_name = "deeplabv3plus_mobilenet_cityscapes.onnx"
# create directory for further converted model
os.makedirs(onnx_model_path, exist_ok=True)
# get full path to the converted model
full_model_path = os.path.join(onnx_model_path, onnx_model_name)
# generate model input
generated_input = Variable(
torch.randn(1, 3, 513, 513)
)
# model export into ONNX format
torch.onnx.export(
original_model,
generated_input,
full_model_path,
verbose=True,
input_names=["input"],
output_names=["output"],
opset_version=11
)
return full_model_path
model = network.modeling.__dict__["deeplabv3plus_mobilenet"](num_classes=19, output_stride=8)
checkpoint = torch.load("best_deeplabv3plus_mobilenet_cityscapes_os16.pth", map_location=torch.device('cpu'))
model.load_state_dict(checkpoint["model_state"])
full_model_path = get_pytorch_onnx_model(model)
注意:我們需要將以上兩個腳本保存并與network文件夾同路徑
2、LabVIEW 調用基于 Pascal VOC2012訓練的deeplabv3+實現圖像語義分割 (deeplabv3+_onnx.vi)
經過實驗發現,opencv dnn因缺少一些算子,所以無法加載deeplabv3+ onnx模型,所以我們選擇使用LabVIEW開放神經網絡交互工具包【ONNX】來加載并推理整個模型,實現語義分割,程序源碼如下:
3、LabVIEW Pascal VOC2012上的分割結果(deeplabv3+_onnx.vi)
4、LabVIEW 調用基于 Cityscapes 訓練的deeplabv3+實現圖像語義分割 (deeplabv3+_onnx_cityscape.vi)
如下圖所示即為程序源碼,我們對比deeplabv3+_onnx.vi,發現其實只需要把模型和待檢測的圖片更換,圖片尺寸比例也做一個修改即可
5、LabVIEW 城市景觀的分割結果(deeplabv3+_onnx_cityscape.vi)
如您想要探討更多關于LabVIEW與人工智能技術,歡迎加入我們的技術交流群:705637299
三、項目源碼及模型下載
歡迎關注微信公眾號: VIRobotics ,回復關鍵字:deepLabv3+ 語義分割源碼 獲取本次分享內容的完整項目源碼及模型。
附加說明
操作系統:Windows10
python:3.6及以上
LabVIEW:2018及以上 64位版本
視覺工具包:techforce_lib_opencv_cpu-1.0.0.73.vip
LabVIEW開放神經網絡交互工具包【ONNX】:virobotics_lib_onnx_cpu-1.0.0.13.vip
總結
以上就是今天要給大家分享的內容。如果有問題可以在評論區里討論。
審核編輯 黃宇
-
LabVIEW
+關注
關注
1974文章
3656瀏覽量
324244 -
人工智能
+關注
關注
1792文章
47387瀏覽量
238900 -
圖像分割
+關注
關注
4文章
182瀏覽量
18018
發布評論請先 登錄
相關推薦
van-自然和醫學圖像的深度語義分割:網絡結構
van-自然和醫學圖像的深度語義分割:網絡結構
聚焦語義分割任務,如何用卷積神經網絡處理語義圖像分割?
DeepLab進行語義分割的研究分析
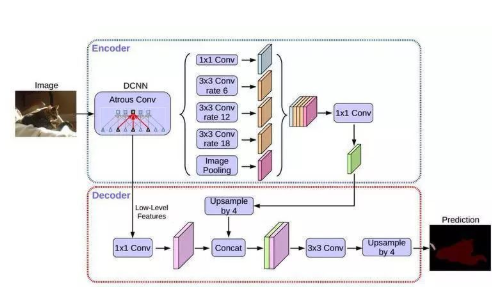
分析總結基于深度神經網絡的圖像語義分割方法
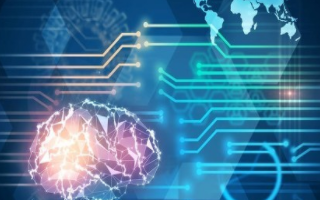
一種改進DeepLabv3+網絡的腸道息肉分割方法
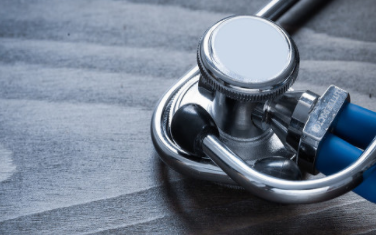
基于深度學習的遙感影像典型要素提取方法
基于圖像語義分割的毛筆筆觸實時生成技術
使用OpenVINO? 部署PaddleSeg模型庫中的DeepLabV3+模型
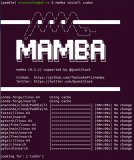
輕松學Pytorch之Deeplabv3推理
圖像分割與語義分割中的CNN模型綜述
圖像語義分割的實用性是什么
常見人體姿態評估顯示方式的兩種方式
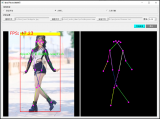
評論