回憶一下圖 7.2.1中的卷積示例。輸入的高度和寬度均為 3,卷積核的高度和寬度均為 2,從而產(chǎn)生具有維度的輸出表示2×2. 假設(shè)輸入形狀是 nh×nw卷積核形狀為 kh×kw,輸出形狀將是 (nh?kh+1)×(nw?kw+1):我們只能將卷積核移動到它用完像素以應(yīng)用卷積為止。
在下文中,我們將探索許多技術(shù),包括填充和跨步卷積,它們可以更好地控制輸出的大小。作為動機,請注意,由于內(nèi)核的寬度和高度通常大于1,在應(yīng)用許多連續(xù)的卷積之后,我們往往會得到比輸入小得多的輸出。如果我們從一個240×240像素圖像,10層層5×5卷積將圖像縮小為200×200像素,切片30%的圖像,并用它抹掉原始圖像邊界上的任何有趣信息。填充是處理此問題的最流行的工具。在其他情況下,我們可能希望大幅降低維數(shù),例如,如果我們發(fā)現(xiàn)原始輸入分辨率很笨重。跨步卷積是一種流行的技術(shù),可以在這些情況下提供幫助。
import torch from torch import nn
from mxnet import np, npx from mxnet.gluon import nn npx.set_np()
import jax from flax import linen as nn from jax import numpy as jnp from d2l import jax as d2l
No GPU/TPU found, falling back to CPU. (Set TF_CPP_MIN_LOG_LEVEL=0 and rerun for more info.)
import tensorflow as tf
7.3.1. 填充
如上所述,應(yīng)用卷積層時的一個棘手問題是我們往往會丟失圖像周邊的像素。考慮 圖 7.3.1,該圖將像素利用率描述為卷積核大小和圖像內(nèi)位置的函數(shù)。角落里的像素幾乎沒有被使用。
圖 7.3.1尺寸卷積的像素利用1×1, 2×2, 和3×3分別。
由于我們通常使用小內(nèi)核,對于任何給定的卷積,我們可能只會丟失幾個像素,但是當(dāng)我們應(yīng)用許多連續(xù)的卷積層時,這可能會累加起來。這個問題的一個直接解決方案是在輸入圖像的邊界周圍添加額外的填充像素,從而增加圖像的有效尺寸。通常,我們將額外像素的值設(shè)置為零。在 圖 7.3.2中,我們填充一個3×3輸入,將其大小增加到5×5. 相應(yīng)的輸出然后增加到4×4矩陣。陰影部分是第一個輸出元素以及用于輸出計算的輸入和內(nèi)核張量元素:0×0+0×1+0×2+0×3=0.
圖 7.3.2帶填充的二維互相關(guān)。
一般來說,如果我們總共添加ph填充行(大約一半在頂部,一半在底部)和總共pw填充列(大約一半在左邊,一半在右邊),輸出形狀將是
(7.3.1)(nh?kh+ph+1)×(nw?kw+pw+1).
這意味著輸出的高度和寬度將增加 ph和pw, 分別。
在許多情況下,我們會想要設(shè)置ph=kh?1和 pw=kw?1給輸入和輸出相同的高度和寬度。這樣在構(gòu)建網(wǎng)絡(luò)時更容易預(yù)測每一層的輸出形狀。假如說kh這里很奇怪,我們會墊ph/2高度兩側(cè)的行。如果 kh是偶數(shù),一種可能是填充 ?ph/2?輸入頂部的行和 ?ph/2?底部的行。我們將以相同的方式填充寬度的兩側(cè)。
CNN 通常使用具有奇數(shù)高度和寬度值的卷積核,例如 1、3、5 或 7。選擇奇數(shù)核大小的好處是我們可以保留維度,同時在頂部和底部填充相同數(shù)量的行,并且左右的列數(shù)相同。
此外,這種使用奇數(shù)內(nèi)核和填充來精確保持維度的做法提供了文書上的好處。對于任意一個二維張量X,當(dāng)核的大小為奇數(shù),且各邊的padding行數(shù)和列數(shù)相同時,產(chǎn)生與輸入等高等寬的輸出,我們知道輸出是通過cross計算的-輸入和卷積核與以 為中心的窗口的相關(guān)性。Y[i, j]X[i, j]
在下面的示例中,我們創(chuàng)建了一個二維卷積層,其高度和寬度均為 3,并在所有邊上應(yīng)用 1 個像素的填充。給定一個高度和寬度為 8 的輸入,我們發(fā)現(xiàn)輸出的高度和寬度也為 8。
# We define a helper function to calculate convolutions. It initializes the # convolutional layer weights and performs corresponding dimensionality # elevations and reductions on the input and output def comp_conv2d(conv2d, X): # (1, 1) indicates that batch size and the number of channels are both 1 X = X.reshape((1, 1) + X.shape) Y = conv2d(X) # Strip the first two dimensions: examples and channels return Y.reshape(Y.shape[2:]) # 1 row and column is padded on either side, so a total of 2 rows or columns # are added conv2d = nn.LazyConv2d(1, kernel_size=3, padding=1) X = torch.rand(size=(8, 8)) comp_conv2d(conv2d, X).shape
torch.Size([8, 8])
# We define a helper function to calculate convolutions. It initializes # the convolutional layer weights and performs corresponding dimensionality # elevations and reductions on the input and output def comp_conv2d(conv2d, X): conv2d.initialize() # (1, 1) indicates that batch size and the number of channels are both 1 X = X.reshape((1, 1) + X.shape) Y = conv2d(X) # Strip the first two dimensions: examples and channels return Y.reshape(Y.shape[2:]) # 1 row and column is padded on either side, so a total of 2 rows or columns are added conv2d = nn.Conv2D(1, kernel_size=3, padding=1) X = np.random.uniform(size=(8, 8)) comp_conv2d(conv2d, X).shape
(8, 8)
# We define a helper function to calculate convolutions. It initializes # the convolutional layer weights and performs corresponding dimensionality # elevations and reductions on the input and output def comp_conv2d(conv2d, X): # (1, X.shape, 1) indicates that batch size and the number of channels are both 1 key = jax.random.PRNGKey(d2l.get_seed()) X = X.reshape((1,) + X.shape + (1,)) Y, _ = conv2d.init_with_output(key, X) # Strip the dimensions: examples and channels return Y.reshape(Y.shape[1:3]) # 1 row and column is padded on either side, so a total of 2 rows or columns are added conv2d = nn.Conv(1, kernel_size=(3, 3), padding='SAME') X = jax.random.uniform(jax.random.PRNGKey(d2l.get_seed()), shape=(8, 8)) comp_conv2d(conv2d, X).shape
(8, 8)
# We define a helper function to calculate convolutions. It initializes # the convolutional layer weights and performs corresponding dimensionality # elevations and reductions on the input and output def comp_conv2d(conv2d, X): # (1, 1) indicates that batch size and the number of channels are both 1 X = tf.reshape(X, (1, ) + X.shape + (1, )) Y = conv2d(X) # Strip the first two dimensions: examples and channels return tf.reshape(Y, Y.shape[1:3]) # 1 row and column is padded on either side, so a total of 2 rows or columns # are added conv2d = tf.keras.layers.Conv2D(1, kernel_size=3, padding='same') X = tf.random.uniform(shape=(8, 8)) comp_conv2d(conv2d, X).shape
TensorShape([8, 8])
當(dāng)卷積核的高和寬不同時,我們可以通過為高和寬設(shè)置不同的填充數(shù),使輸出和輸入具有相同的高和寬。
# We use a convolution kernel with height 5 and width 3. The padding on either # side of the height and width are 2 and 1, respectively conv2d = nn.LazyConv2d(1, kernel_size=(5, 3), padding=(2, 1)) comp_conv2d(conv2d, X).shape
torch.Size([8, 8])
# We use a convolution kernel with height 5 and width 3. The padding on # either side of the height and width are 2 and 1, respectively conv2d = nn.Conv2D(1, kernel_size=(5, 3), padding=(2, 1)) comp_conv2d(conv2d, X).shape
(8, 8)
# We use a convolution kernel with height 5 and width 3. The padding on # either side of the height and width are 2 and 1, respectively conv2d = nn.Conv(1, kernel_size=(5, 3), padding=(2, 1)) comp_conv2d(conv2d, X).shape
(8, 8)
# We use a convolution kernel with height 5 and width 3. The padding on # either side of the height and width are 2 and 1, respectively conv2d = tf.keras.layers.Conv2D(1, kernel_size=(5, 3), padding='same') comp_conv2d(conv2d, X).shape
TensorShape([8, 8])
7.3.2. 步幅
在計算互相關(guān)時,我們從輸入張量左上角的卷積窗口開始,然后將其滑過所有位置,包括向下和向右。在前面的示例中,我們默認(rèn)一次滑動一個元素。然而,有時,無論是為了提高計算效率還是因為我們希望下采樣,我們一次將窗口移動一個以上的元素,跳過中間位置。如果卷積核很大,這是特別有用的,因為它捕獲了大面積的底層圖像。
我們將每張幻燈片遍歷的行數(shù)和列數(shù)稱為 步幅。到目前為止,我們對高度和寬度都使用了 1 的步幅。有時,我們可能想使用更大的步幅。 圖 7.3.3顯示了垂直步長為 3,水平步長為 2 的二維互相關(guān)運算。陰影部分是輸出元素以及用于輸出計算的輸入和內(nèi)核張量元素: 0×0+0×1+1×2+2×3=8, 0×0+6×1+0×2+0×3=6. 我們可以看到,當(dāng)?shù)谝涣械牡诙€元素生成時,卷積窗口向下滑動了三行。當(dāng)生成第一行的第二個元素時,卷積窗口向右滑動兩列。當(dāng)卷積窗口在輸入上繼續(xù)向右滑動兩列時,就沒有輸出了,因為輸入元素?zé)o法填滿窗口(除非我們再添加一列padding)。
圖 7.3.3高度和寬度的步長分別為 3 和 2 的互相關(guān)。
一般來說,當(dāng)高度的步幅為sh寬度的步幅是sw,輸出形狀為
(7.3.2)?(nh?kh+ph+sh)/sh?×?(nw?kw+pw+sw)/sw?.
如果我們設(shè)置ph=kh?1和pw=kw?1, 那么輸出形狀可以簡化為 ?(nh+sh?1)/sh?×?(nw+sw?1)/sw?. 更進一步,如果輸入的高度和寬度可以被高度和寬度的步幅整除,那么輸出形狀將是 (nh/sh)×(nw/sw).
下面,我們將高度和寬度的步幅都設(shè)置為 2,從而將輸入的高度和寬度減半。
conv2d = nn.LazyConv2d(1, kernel_size=3, padding=1, stride=2) comp_conv2d(conv2d, X).shape
torch.Size([4, 4])
conv2d = nn.Conv2D(1, kernel_size=3, padding=1, strides=2) comp_conv2d(conv2d, X).shape
(4, 4)
conv2d = nn.Conv(1, kernel_size=(3, 3), padding=1, strides=2) comp_conv2d(conv2d, X).shape
(4, 4)
conv2d = tf.keras.layers.Conv2D(1, kernel_size=3, padding='same', strides=2) comp_conv2d(conv2d, X).shape
TensorShape([4, 4])
讓我們看一個稍微復(fù)雜一點的例子。
conv2d = nn.LazyConv2d(1, kernel_size=(3, 5), padding=(0, 1), stride=(3, 4)) comp_conv2d(conv2d, X).shape
torch.Size([2, 2])
conv2d = nn.Conv2D(1, kernel_size=(3, 5), padding=(0, 1), strides=(3, 4)) comp_conv2d(conv2d, X).shape
(2, 2)
conv2d = nn.Conv(1, kernel_size=(3, 5), padding=(0, 1), strides=(3, 4)) comp_conv2d(conv2d, X).shape
(2, 2)
conv2d = tf.keras.layers.Conv2D(1, kernel_size=(3,5), padding='valid', strides=(3, 4)) comp_conv2d(conv2d, X).shape
TensorShape([2, 1])
7.3.3. 總結(jié)與討論
填充可以增加輸出的高度和寬度。這通常用于為輸出提供與輸入相同的高度和寬度,以避免不希望的輸出收縮。此外,它確保所有像素的使用頻率相同。通常我們在輸入高度和寬度的兩側(cè)選擇對稱填充。在這種情況下,我們指的是 (ph,pw)填充。最常見的是我們設(shè)置ph=pw,在這種情況下,我們只是聲明我們選擇填充p.
類似的約定適用于步幅。橫步時 sh和垂直步幅swmatch,我們簡單說說strides. 步幅可以降低輸出的分辨率,例如將輸出的高度和寬度降低到僅 1/n輸入的高度和寬度n>1. 默認(rèn)情況下,填充為 0,步幅為 1。
到目前為止,我們討論的所有填充都只是用零擴展圖像。這具有顯著的計算優(yōu)勢,因為它很容易實現(xiàn)。此外,可以將運算符設(shè)計為隱式利用此填充,而無需分配額外的內(nèi)存。同時,它允許 CNN 對圖像中的隱式位置信息進行編碼,只需了解“空白”的位置即可。零填充有很多替代方法。 Alsallakh等人。( 2020 )提供了替代方案的廣泛概述(盡管沒有明確的案例使用非零填充,除非出現(xiàn)偽影)。
7.3.4. 練習(xí)
給定本節(jié)中最后一個具有內(nèi)核大小的代碼示例 (3,5), 填充(0,1), 和大步(3,4), 計算輸出形狀以檢查它是否與實驗結(jié)果一致。
實施鏡像填充,即邊界值被簡單地鏡像以擴展張量的填充。
步幅大于 1 的計算優(yōu)勢是什么?
大于 1 的步幅在統(tǒng)計上有什么好處?
你將如何實現(xiàn)一大步12?它對應(yīng)什么?這什么時候有用?
-
pytorch
+關(guān)注
關(guān)注
2文章
808瀏覽量
13283
發(fā)布評論請先 登錄
相關(guān)推薦
Pytorch模型訓(xùn)練實用PDF教程【中文】
如何往星光2板子里裝pytorch?
CEA R7.3 標(biāo)準(zhǔn),CEA R7.3 標(biāo)準(zhǔn)是什么意思
基于步數(shù)步幅統(tǒng)計的測距方法
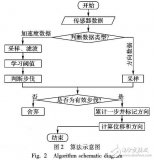
評論