在這篇文章中,我們將討論如何將Keras模型轉(zhuǎn)換為TensorFlow session。
Keras是一個(gè)高級(jí)神經(jīng)網(wǎng)絡(luò)API,它提供了一種簡(jiǎn)單、快速的方式來(lái)構(gòu)建和訓(xùn)練深度學(xué)習(xí)模型。Keras是基于TensorFlow、Theano或CNTK等底層計(jì)算框架構(gòu)建的。TensorFlow是一個(gè)開(kāi)源的機(jī)器學(xué)習(xí)框架,由Google Brain團(tuán)隊(duì)開(kāi)發(fā)。它提供了一種靈活的方式來(lái)構(gòu)建和訓(xùn)練深度學(xué)習(xí)模型,支持多種硬件平臺(tái)。
- Keras模型和TensorFlow session的關(guān)系
Keras模型是一個(gè)高級(jí)抽象,它隱藏了底層的TensorFlow細(xì)節(jié)。當(dāng)你使用Keras構(gòu)建模型時(shí),實(shí)際上是在構(gòu)建一個(gè)TensorFlow計(jì)算圖。Keras模型的權(quán)重和偏置是TensorFlow變量,模型的前向傳播和反向傳播都是TensorFlow操作。
TensorFlow session是TensorFlow中的一個(gè)執(zhí)行環(huán)境,它負(fù)責(zé)執(zhí)行計(jì)算圖中的操作。在TensorFlow 1.x版本中,你需要顯式地創(chuàng)建和關(guān)閉session。但在TensorFlow 2.x版本中,session的概念已經(jīng)被簡(jiǎn)化,你可以直接使用TensorFlow API來(lái)執(zhí)行操作。
- 為什么需要將Keras模型轉(zhuǎn)換為T(mén)ensorFlow session
在某些情況下,你可能需要將Keras模型轉(zhuǎn)換為T(mén)ensorFlow session,以便更好地控制模型的執(zhí)行。例如,你可能需要在特定的硬件上運(yùn)行模型,或者需要使用TensorFlow的一些高級(jí)特性,如分布式訓(xùn)練、自定義訓(xùn)練循環(huán)等。
- 如何將Keras模型轉(zhuǎn)換為T(mén)ensorFlow session
在TensorFlow 2.x版本中,Keras模型已經(jīng)與TensorFlow session緊密集成。你可以直接使用Keras模型的compile
、fit
、evaluate
和predict
等方法來(lái)訓(xùn)練和評(píng)估模型。但在某些情況下,你可能需要顯式地創(chuàng)建一個(gè)TensorFlow session來(lái)執(zhí)行模型。
以下是一個(gè)示例,展示了如何將Keras模型轉(zhuǎn)換為T(mén)ensorFlow session:
import tensorflow as tf
from tensorflow import keras
# 創(chuàng)建一個(gè)Keras模型
model = keras.Sequential([
keras.layers.Dense(64, activation='relu', input_shape=(32,)),
keras.layers.Dense(64, activation='relu'),
keras.layers.Dense(10, activation='softmax')
])
# 編譯模型
model.compile(optimizer='adam',
loss='sparse_categorical_crossentropy',
metrics=['accuracy'])
# 準(zhǔn)備數(shù)據(jù)
x_train = ... # 訓(xùn)練數(shù)據(jù)
y_train = ... # 訓(xùn)練標(biāo)簽
# 創(chuàng)建一個(gè)TensorFlow session
with tf.compat.v1.Session() as sess:
# 將Keras模型轉(zhuǎn)換為T(mén)ensorFlow session
k_sess = tf.compat.v1.keras.backend.get_session()
# 訓(xùn)練模型
model.fit(x_train, y_train, epochs=10, batch_size=32, session=k_sess)
# 評(píng)估模型
loss, accuracy = model.evaluate(x_train, y_train, session=k_sess)
print("Loss:", loss)
print("Accuracy:", accuracy)
- 轉(zhuǎn)換過(guò)程中可能遇到的問(wèn)題和解決方案
在將Keras模型轉(zhuǎn)換為T(mén)ensorFlow session的過(guò)程中,你可能會(huì)遇到一些問(wèn)題。以下是一些常見(jiàn)的問(wèn)題及其解決方案:
5.1. 在TensorFlow 2.x版本中使用TensorFlow 1.x版本的API
在TensorFlow 2.x版本中,一些TensorFlow 1.x版本的API已經(jīng)被棄用或更改。如果你的代碼中使用了這些API,你可能需要使用tf.compat.v1
模塊來(lái)訪問(wèn)它們。例如,tf.Session
在TensorFlow 2.x版本中已經(jīng)被棄用,你可以使用tf.compat.v1.Session
來(lái)替代。
5.2. 在TensorFlow session中使用Keras模型的權(quán)重
在TensorFlow session中,你可能需要訪問(wèn)Keras模型的權(quán)重。你可以使用model.get_weights()
方法來(lái)獲取權(quán)重,然后使用tf.Variable
來(lái)創(chuàng)建TensorFlow變量。以下是一個(gè)示例:
# 獲取Keras模型的權(quán)重
weights = model.get_weights()
# 創(chuàng)建TensorFlow變量
tf_weights = [tf.Variable(w) for w in weights]
# 在TensorFlow session中使用權(quán)重
with tf.compat.v1.Session() as sess:
sess.run(tf.compat.v1.global_variables_initializer(tf_weights))
# 使用tf_weights進(jìn)行操作
5.3. 在TensorFlow session中使用Keras模型的損失函數(shù)和優(yōu)化器
在TensorFlow session中,你可能需要使用Keras模型的損失函數(shù)和優(yōu)化器。你可以使用model.loss_functions
和model.optimizer
屬性來(lái)訪問(wèn)它們。以下是一個(gè)示例:
# 獲取Keras模型的損失函數(shù)和優(yōu)化器
loss_fns = model.loss_functions
optimizer = model.optimizer
-
模型
+關(guān)注
關(guān)注
1文章
3243瀏覽量
48836 -
深度學(xué)習(xí)
+關(guān)注
關(guān)注
73文章
5503瀏覽量
121157 -
tensorflow
+關(guān)注
關(guān)注
13文章
329瀏覽量
60535 -
keras
+關(guān)注
關(guān)注
2文章
20瀏覽量
6085
發(fā)布評(píng)論請(qǐng)先 登錄
相關(guān)推薦
Keras之ML~P:基于Keras中建立的回歸預(yù)測(cè)的神經(jīng)網(wǎng)絡(luò)模型
RK3399Pro入門(mén)教程(4)從Tensorflow.Keras到RKNN
STM32CubeAI-Keras具有多個(gè)輸入的順序模型給出內(nèi)部錯(cuò)誤是什么原因?如何處理?
為什么無(wú)法加載keras模型?
keras順序模型與函數(shù)式模型
keras可視化介紹
Keras搭建神經(jīng)網(wǎng)絡(luò)的一般步驟
Keras和TensorFlow究竟哪個(gè)會(huì)更好?
TensorFlow和Keras哪個(gè)更好用?
深入了解TensorFlow隨附的此版Keras將能為您實(shí)現(xiàn)哪些功能
最新tf.keras指南,TensorFlow官方出品
基于TensorFlow和Keras的圖像識(shí)別
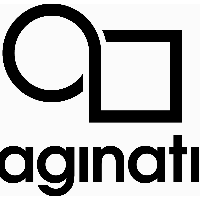
評(píng)論