深度學(xué)習(xí)領(lǐng)域正在迅速發(fā)展,在處理各種類型的任務(wù)中,預(yù)訓(xùn)練模型變得越來越重要。Keras 以其用戶友好型 API 和對(duì)易用性的重視而聞名,始終處于這一動(dòng)向的前沿。Keras 擁有專用的內(nèi)容庫,如用于文本模型的 KerasNLP 和用于計(jì)算機(jī)視覺模型的 KerasCV。
然而,隨著模型使各模態(tài)之間的界限越來越模糊(想象一下強(qiáng)大的聊天 LLM 具有圖像輸入功能或是在視覺任務(wù)中利用文本編碼器),維持這些獨(dú)立的領(lǐng)域變得不那么實(shí)際。NLP 和 CV 之間的區(qū)別可能會(huì)阻礙真正多模態(tài)模型的發(fā)展和部署,從而導(dǎo)致冗余的工作和碎片化的用戶體驗(yàn)。
為了解決這個(gè)問題,我們很高興地宣布 Keras 生態(tài)系統(tǒng)迎來重大變革:隆重推出 KerasHub,一個(gè)統(tǒng)一、全面的預(yù)訓(xùn)練模型庫,簡(jiǎn)化了對(duì)前沿 NLP 和 CV 架構(gòu)的訪問。KerasHub 是一個(gè)中央存儲(chǔ)庫,您可以在穩(wěn)定且熟悉的 Keras 框架內(nèi)無縫探索和使用最先進(jìn)的模型,例如用于文本分析的 BERT 以及用于圖像分類的 EfficientNet。
KerasHub https://keras.io/keras_hub/
統(tǒng)一的開發(fā)者體驗(yàn)
這種統(tǒng)一不僅簡(jiǎn)化了對(duì)模型的探索和使用,還有助于打造更具凝聚力的生態(tài)系統(tǒng)。通過 KerasHub,您可以利用高級(jí)功能,例如輕松的發(fā)布和共享模型、用于優(yōu)化資源效率的 LoRA 微調(diào)、用于優(yōu)化性能的量化,以及用于處理大規(guī)模數(shù)據(jù)集的強(qiáng)大多主機(jī)訓(xùn)練,所有這些功能都適用于各種模態(tài)。這標(biāo)志著在普及強(qiáng)大的 AI 工具以及加速開發(fā)創(chuàng)新型多模態(tài)應(yīng)用方面邁出了重要一步。
KerasHub 入門步驟
首先在您的系統(tǒng)上安裝 KerasHub,您可以在其中探索大量現(xiàn)成的模型和主流架構(gòu)的不同實(shí)現(xiàn)方式。然后,您就可以輕松地將這些預(yù)訓(xùn)練的模型加載并整合到自己的項(xiàng)目中,并根據(jù)您的具體需求對(duì)其進(jìn)行微調(diào),以獲得最佳性能。
現(xiàn)成的模型 https://keras.io/api/keras_hub/models/
安裝 KerasHub
要安裝帶有 Keras 3 的 KerasHub 最新版本,只需運(yùn)行以下代碼:
$ pip install --upgrade keras-hub現(xiàn)在,您可以開始探索可用的模型。使用 Keras 3 開始工作的標(biāo)準(zhǔn)環(huán)境設(shè)置在開始使用 KerasHub 時(shí)并不需要任何改變:
import os # Define the Keras 3 backend you want to use - "jax", "tensorflow" or "torch" os.environ["KERAS_BACKEND"] = "jax" # Import Keras 3 and KerasHub modules import keras import keras_hub
通過 KerasHub 使用
計(jì)算機(jī)視覺和自然語言模型
現(xiàn)在,您可以通過 KerasHub 訪問和使用 Keras 3 生態(tài)系統(tǒng)中的模型。以下是一些示例:
Gemma
Gemma 是由 Google 開發(fā)的一系列前沿且易于使用的開放模型。依托于與 Gemini 模型相同的研究和技術(shù),Gemma 的基礎(chǔ)模型在各種文本生成任務(wù)中表現(xiàn)出色,包括回答問題、總結(jié)信息以及進(jìn)行邏輯推理。此外,您還可以針對(duì)特定需求自定義模型。
Gemma https://ai.google.dev/gemma/docs/base
在此示例中,您可以使用 Keras 和 KerasHub 加載并開始使用 Gemma 2 2B 參數(shù)生成內(nèi)容。有關(guān) Gemma 變體的更多詳細(xì)信息,請(qǐng)查看 Kaggle 上的 Gemma 模型卡。
# Load Gemma 2 2B preset from Kaggle models gemma_lm = keras_hub.models.GemmaCausalLM.from_preset("gemma_2b_en") # Start generating contents with Gemma 2 2B gemma_lm.generate("Keras is a", max_length=32)
Gemma 模型卡 https://www.kaggle.com/models/google/gemma/
PaliGemma
PaliGemma 是一款緊湊型的開放模型,可以理解圖像和文本。PaliGemma 從 PaLI-3 中汲取靈感,以 SigLIP 視覺模型和 Gemma 語言模型等開源組件為基礎(chǔ),可以針對(duì)有關(guān)圖像的問題提供詳細(xì)且富有洞察力的答案。因此,該模型可以更深入地了解視覺內(nèi)容,從而實(shí)現(xiàn)諸多功能,例如為圖像和短視頻生成描述、識(shí)別對(duì)象甚至理解圖像中的文本。
import os # Define the Keras 3 backend you want to use - "jax", "tensorflow" or "torch" os.environ["KERAS_BACKEND"] = "jax" # Import Keras 3 and KerasHub modules import keras import keras_hub from keras.utils import get_file, load_img, img_to_array # Import PaliGemma 3B fine tuned with 224x224 images pali_gemma_lm = keras_hub.models.PaliGemmaCausalLM.from_preset( "pali_gemma_3b_mix_224" ) # Download a test image and prepare it for usage with KerasHub url = 'https://storage.googleapis.com/keras-cv/models/paligemma/cow_beach_1.png' img_path = get_file(origin=url) img = img_to_array(load_img(image_path)) # Create the prompt with the question about the image prompt = 'answer where is the cow standing?' # Generate the contents with PaliGemma output = pali_gemma_lm.generate( inputs={ "images": img, "prompts": prompt, } )
PaliGemma https://ai.google.dev/gemma/docs/paligemma
PaLI-3 https://arxiv.org/abs/2310.09199
SigLIP 視覺模型 https://arxiv.org/abs/2303.15343
Gemma 語言模型 https://arxiv.org/abs/2403.08295
有關(guān) Keras 3 上可用的預(yù)訓(xùn)練模型的更多詳細(xì)信息,請(qǐng)?jiān)?Kaggle 上查看 Keras 中的模型列表。
Kaggle 上查看 Keras 中的模型列表 https://www.kaggle.com/organizations/keras/models
Stability.ai Stable Diffusion 3
您也可以使用計(jì)算機(jī)視覺模型。例如,您可以通過 KerasHub 使用 stability.ai Stable Diffusion 3:
from PIL import Image from keras.utils import array_to_img from keras_hub.models import StableDiffusion3TextToImage text_to_image = StableDiffusion3TextToImage.from_preset( "stable_diffusion_3_medium", height=1024, width=1024, dtype="float16", ) # Generate images with SD3 image = text_to_image.generate( "photograph of an astronaut riding a horse, detailed, 8k", ) # Display the generated image img = array_to_img(image) img
Stable Diffusion 3 https://stability.ai/news/stable-diffusion-3
有關(guān) Keras 3 上可用的預(yù)訓(xùn)練計(jì)算機(jī)視覺模型的更多詳細(xì)信息,請(qǐng)查看 Keras 中的模型列表。
Keras 中的模型列表 https://keras.io/api/keras_hub/models/
對(duì)于 KerasNLP 開發(fā)者而言,
有哪些變化?
從 KerasNLP 到 KerasHub 的過渡是一個(gè)簡(jiǎn)單的過程。只需要將 import 語句從 keras_nlp 更新為 keras_hub。
示例: 以前,您可能需要導(dǎo)入 keras_nlp 才能使用 BERT 模型,如下所示
import keras_nlp # Load a BERT model classifier = keras_nlp.models.BertClassifier.from_preset( "bert_base_en_uncased", num_classes=2, )現(xiàn)在,您只需調(diào)整 import,即可使用 KerasHub:
import keras_hub # Load a BERT model classifier = keras_hub.models.BertClassifier.from_preset( "bert_base_en_uncased", num_classes=2, )
對(duì)于 KerasCV 開發(fā)者而言,
有哪些變化?
如果您當(dāng)前是 KerasCV 用戶,更新到 KerasHub 能夠?yàn)槟鷰硪韵潞锰?
簡(jiǎn)化模型加載:KerasHub 為加載模型提供了統(tǒng)一的 API,如果您同時(shí)使用 KerasCV 和 KerasNLP,這可以簡(jiǎn)化您的代碼。
框架靈活性:如果您有興趣探索 JAX 或 PyTorch 等不同框架,KerasHub 可以讓您更輕松地將這些框架與 KerasCV 和 KerasNLP 模型結(jié)合起來使用。
集中式存儲(chǔ)庫:借助 KerasHub 的統(tǒng)一模型存儲(chǔ)庫,您可以更輕松地查找和訪問模型,未來還可以在其中添加新架構(gòu)。
如何使我的代碼適配 KerasHub?
模型
目前,我們正在將 KerasCV 模型遷移到 KerasHub。雖然大多數(shù)模型已經(jīng)可用,但有些仍在遷移中。請(qǐng)注意,Centerpillar 模型不會(huì)被遷移。您應(yīng)該能夠在 KerasHub 使用任何視覺模型,方法如下:
import keras_hub # Load a model using preset Model = keras_hub.models..from_preset('preset_name`) # or load a custom model by specifying the backbone and preprocessor Model=keras_hub.models. (backbone=backbone,preprocessor=preprocessor)
Centerpillar https://www.kaggle.com/models/keras/centerpillar
KerasHub 為 KerasCV 開發(fā)者帶來了激動(dòng)人心的新功能,提供了更高的靈活性和擴(kuò)展能力。其中包括:
內(nèi)置預(yù)處理
每個(gè)模型都配備了一個(gè)定制的預(yù)處理器,用于處理包括調(diào)整大小、重新縮放等常規(guī)任務(wù),從而簡(jiǎn)化您的工作流程。 在此之前,預(yù)處理輸入是在向模型提供輸入之前手動(dòng)執(zhí)行的。
# Preprocess inputs for example def preprocess_inputs(image, label): # Resize rescale or do more preprocessing on inputs return preprocessed_inputs backbone = keras_cv.models.ResNet50V2Backbone.from_preset( "resnet50_v2_imagenet", ) model = keras_cv.models.ImageClassifier( backbone=backbone, num_classes=4, ) output = model(preprocessed_input)目前,任務(wù)模型的預(yù)處理已集成到現(xiàn)成的預(yù)設(shè)中。預(yù)處理器會(huì)對(duì)輸入進(jìn)行預(yù)處理,對(duì)樣本圖像進(jìn)行大小調(diào)整和重新縮放。預(yù)處理器是任務(wù)模型的內(nèi)在組件。盡管如此,開發(fā)者還是可以選擇使用個(gè)性化的預(yù)處理器。
classifier = keras_hub.models.ImageClassifier.from_preset('resnet_18_imagenet') classifier.predict(inputs)
損失函數(shù)
與增強(qiáng)層類似,以前 KerasCV 中的損失函數(shù)現(xiàn)在可在 Keras 中通過 keras.losses.
import keras import keras_cv keras_cv.losses.FocalLoss( alpha=0.25, gamma=2, from_logits=False, label_smoothing=0, **kwargs )
FocalLoss 函數(shù) https://keras.io/api/keras_cv/losses/focal_loss/
您只需調(diào)整損失函數(shù)定義代碼,使用 keras.losses 而不是 keras_cv.losses:
import keras keras.losses.FocalLoss( alpha=0.25, gamma=2, from_logits=False, label_smoothing=0, **kwargs )
-
模型
+關(guān)注
關(guān)注
1文章
3243瀏覽量
48836 -
深度學(xué)習(xí)
+關(guān)注
關(guān)注
73文章
5503瀏覽量
121157 -
nlp
+關(guān)注
關(guān)注
1文章
488瀏覽量
22035
原文標(biāo)題:Keras Hub,您的一站式預(yù)訓(xùn)練模型庫
文章出處:【微信號(hào):Google_Developers,微信公眾號(hào):谷歌開發(fā)者】歡迎添加關(guān)注!文章轉(zhuǎn)載請(qǐng)注明出處。
發(fā)布評(píng)論請(qǐng)先 登錄
相關(guān)推薦
開源AI模型庫是干嘛的
什么是大模型、大模型是怎么訓(xùn)練出來的及大模型作用
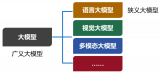
從零開始訓(xùn)練一個(gè)大語言模型需要投資多少錢?

直播預(yù)約 |數(shù)據(jù)智能系列講座第4期:預(yù)訓(xùn)練的基礎(chǔ)模型下的持續(xù)學(xué)習(xí)
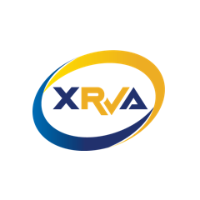
評(píng)論