在此之前,我們介紹過用于自動(dòng)微分的 TensorFlow API - 自動(dòng)微分,優(yōu)化機(jī)器學(xué)習(xí)模型的關(guān)鍵技術(shù),這是機(jī)器學(xué)習(xí)的基本構(gòu)建塊。在今天的教程中,我們將使用先前教程中介紹的 TensorFlow 基礎(chǔ)來進(jìn)行一些簡單的機(jī)器學(xué)習(xí)。
TensorFlow 還包括一個(gè)更高級(jí)別的神經(jīng)網(wǎng)絡(luò) API(tf.keras),它提供了有用的抽象來減少樣板。我們強(qiáng)烈建議那些使用神經(jīng)網(wǎng)絡(luò)的人使用更高級(jí)別的 API。但是,在這個(gè)簡短的教程中我們將從神經(jīng)網(wǎng)絡(luò)訓(xùn)練的基本原理來建立一個(gè)堅(jiān)實(shí)的基礎(chǔ)。
設(shè)置
import tensorflow as tftf.enable_eager_execution()
變量
TensorFlow 中的張量是不可變的無狀態(tài)對(duì)象。然而,機(jī)器學(xué)習(xí)模型需要具有可變的狀態(tài):隨著模型的訓(xùn)練,計(jì)算預(yù)測的相同代碼應(yīng)該隨著時(shí)間的推移而表現(xiàn)不同(希望具有較低的損失!)。要表示在計(jì)算過程中需要改變的狀態(tài),事實(shí)上您可以選擇依賴 Python 這種有狀態(tài)的編程語言:
# Using python statex = tf.zeros([10, 10])x += 2 # This is equivalent to x = x + 2, which does not mutate the original # value of xprint(x)
tf.Tensor([[2。2. 2. 2. 2. 2. 2. 2. 2. 2.] [2。2. 2. 2. 2. 2. 2. 2. 2. 2.] [2。2. 2. 2. 2. 2. 2. 2. 2. 2.] [2。2. 2. 2. 2. 2. 2. 2. 2. 2.] [2。2. 2. 2. 2. 2. 2. 2. 2. 2.] [2。2. 2. 2. 2. 2. 2. 2. 2. 2.] [2。2. 2. 2. 2. 2. 2. 2. 2. 2.] [2。2. 2. 2. 2. 2. 2. 2. 2. 2.] [2。2. 2. 2. 2. 2. 2. 2. 2. 2.] [2。2. 2. 2. 2. 2. 2. 2. 2. 2.]],shape =(10,10),dtype = float32)
但是,TensorFlow 內(nèi)置了有狀態(tài)操作,這些操作通常比您所用的低級(jí) Python 表示更易于使用。例如,為了表示模型中的權(quán)重,使用 TensorFlow 變量通常是方便有效的。
變量是一個(gè)存儲(chǔ)值的對(duì)象,當(dāng)在 TensorFlow 計(jì)算中使用時(shí),它將隱式地從該存儲(chǔ)值中讀取。有些操作(如:tf.assign_sub,tf.scatter_update 等)會(huì)操縱存儲(chǔ)在 TensorFlow 變量中的值。
v = tf.Variable(1.0)assert v.numpy() == 1.0# Re-assign the valuev.assign(3.0)assert v.numpy() == 3.0# Use `v` in a TensorFlow operation like tf.square() and reassignv.assign(tf.square(v))assert v.numpy() == 9.0
使用變量的計(jì)算在計(jì)算梯度時(shí)自動(dòng)跟蹤。對(duì)于表示嵌入式的變量,TensorFlow 默認(rèn)會(huì)進(jìn)行稀疏更新,這樣可以提高計(jì)算效率和內(nèi)存效率。
使用變量也是一種快速讓代碼的讀者知道這段狀態(tài)是可變的方法。
示例:擬合線性模型
現(xiàn)在讓我們把目前掌握的幾個(gè)概念 — 張量、梯度帶、變量 — 應(yīng)用到構(gòu)建和訓(xùn)練一個(gè)簡單模型中去。這通常涉及幾個(gè)步驟:
1.定義模型。
2.定義損失函數(shù)。
3.獲取訓(xùn)練數(shù)據(jù)。
4.運(yùn)行訓(xùn)練數(shù)據(jù)并使用 “優(yōu)化器” 調(diào)整變量以匹配數(shù)據(jù)。
在本教程中,我們將介紹一個(gè)簡單線性模型的簡單示例:f(x) = x * W + b,它有兩個(gè)變量 —W 和 b。此外,我們將綜合數(shù)據(jù),以便訓(xùn)練好的模型具有 W = 3.0 和 b = 2.0。
定義模型
讓我們定義一個(gè)簡單的類來封裝變量和計(jì)算。
class Model(object): def __init__(self): # Initialize variable to (5.0, 0.0) # In practice, these should be initialized to random values. self.W = tf.Variable(5.0) self.b = tf.Variable(0.0) def __call__(self, x): return self.W * x + self.b model = Model()assert model(3.0).numpy() == 15.0
定義損失函數(shù)
損失函數(shù)測量給定輸入的模型輸出與期望輸出的匹配程度。讓我們使用標(biāo)準(zhǔn)的 L2 損失。
def loss(predicted_y, desired_y): return tf.reduce_mean(tf.square(predicted_y - desired_y))
獲取訓(xùn)練數(shù)據(jù)
讓我們用一些噪音(noise)合成訓(xùn)練數(shù)據(jù)。
TRUE_W = 3.0TRUE_b = 2.0NUM_EXAMPLES = 1000inputs = tf.random_normal(shape=[NUM_EXAMPLES])noise = tf.random_normal(shape=[NUM_EXAMPLES])outputs = inputs * TRUE_W + TRUE_b + noise
在我們訓(xùn)練模型之前,讓我們想象一下模型現(xiàn)在的位置。我們將用紅色繪制模型的預(yù)測,用藍(lán)色繪制訓(xùn)練數(shù)據(jù)。
import matplotlib.pyplot as pltplt.scatter(inputs, outputs, c='b')plt.scatter(inputs, model(inputs), c='r')plt.show()print('Current loss: '),print(loss(model(inputs), outputs).numpy())
Current loss:
7.92897
定義訓(xùn)練循環(huán)
我們現(xiàn)在有了網(wǎng)絡(luò)和培訓(xùn)數(shù)據(jù)。我們來訓(xùn)練一下,使用訓(xùn)練數(shù)據(jù)更新模型的變量 ( W 和 b),以便使用梯度下降減少損失。在 tf.train.Optimizer 實(shí)現(xiàn)中有許多梯度下降方案的變體。我們強(qiáng)烈建議使用這種實(shí)現(xiàn),但本著從基本原理出發(fā)的精神,在這個(gè)特定的例子中,我們將自己實(shí)現(xiàn)基本的數(shù)學(xué)。
def train(model, inputs, outputs, learning_rate): with tf.GradientTape() as t: current_loss = loss(model(inputs), outputs) dW, db = t.gradient(current_loss, [model.W, model.b]) model.W.assign_sub(learning_rate * dW) model.b.assign_sub(learning_rate * db)
最后,讓我們反復(fù)運(yùn)行訓(xùn)練數(shù)據(jù),看看 W 和 b 是如何發(fā)展的。
model = Model()# Collect the history of W-values and b-values to plot laterWs, bs = [], []epochs = range(10)for epoch in epochs: Ws.append(model.W.numpy()) bs.append(model.b.numpy()) current_loss = loss(model(inputs), outputs) train(model, inputs, outputs, learning_rate=0.1) print('Epoch %2d: W=%1.2f b=%1.2f, loss=%2.5f' % (epoch, Ws[-1], bs[-1], current_loss))# Let's plot it allplt.plot(epochs, Ws, 'r', epochs, bs, 'b')plt.plot([TRUE_W] * len(epochs), 'r--', [TRUE_b] * len(epochs), 'b--')plt.legend(['W', 'b', 'true W', 'true_b'])plt.show()
Epoch 0: W=5.00 b=0.00, loss=7.92897Epoch 1: W=4.64 b=0.35, loss=5.61977Epoch 2: W=4.35 b=0.64, loss=4.07488Epoch 3: W=4.11 b=0.88, loss=3.04133Epoch 4: W=3.91 b=1.07, loss=2.34987Epoch 5: W=3.75 b=1.23, loss=1.88727Epoch 6: W=3.62 b=1.36, loss=1.57779Epoch 7: W=3.51 b=1.47, loss=1.37073Epoch 8: W=3.42 b=1.55, loss=1.23221Epoch 9: W=3.35 b=1.62, loss=1.13954
下一步
在本教程中,我們介紹了變量 Variables,使用了到目前為止討論的 TensorFlow 基本原理構(gòu)建并訓(xùn)練了一個(gè)簡單的線性模型。
從理論上講,這幾乎是您使用 TensorFlow 進(jìn)行機(jī)器學(xué)習(xí)研究所需要的全部內(nèi)容。在實(shí)踐中,特別是對(duì)于神經(jīng)網(wǎng)絡(luò),更高級(jí)別的 APItf.keras 會(huì)更方便,因?yàn)樗峁└呒?jí)別的構(gòu)建塊(稱為 “層”),保存和恢復(fù)狀態(tài)的實(shí)用程序,一套損失函數(shù),一套優(yōu)化策略等等。
-
機(jī)器學(xué)習(xí)
+關(guān)注
關(guān)注
66文章
8418瀏覽量
132634 -
tensorflow
+關(guān)注
關(guān)注
13文章
329瀏覽量
60536
原文標(biāo)題:帶你使用 TensorFlow 進(jìn)行機(jī)器學(xué)習(xí)研究
文章出處:【微信號(hào):tensorflowers,微信公眾號(hào):Tensorflowers】歡迎添加關(guān)注!文章轉(zhuǎn)載請(qǐng)注明出處。
發(fā)布評(píng)論請(qǐng)先 登錄
相關(guān)推薦
如何使用TensorFlow構(gòu)建機(jī)器學(xué)習(xí)模型
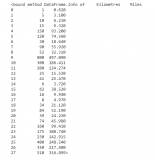
關(guān)于 TensorFlow
谷歌深度學(xué)習(xí)插件tensorflow
干貨!教你怎么搭建TensorFlow深度學(xué)習(xí)開發(fā)環(huán)境!
tensorflow機(jī)器學(xué)習(xí)日志
TensorFlow的特點(diǎn)和基本的操作方式
labview+yolov4+tensorflow+openvion深度學(xué)習(xí)
TensorFlow的框架結(jié)構(gòu)解析
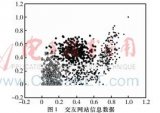
評(píng)論